Partnering with causaLens – 5 Customer Stories and their perceived Value Propositions
Fortune 1000s decide to partner with causaLens in order to adopt & scale Causal AI across their organisation in the fastest & most cost-efficient way possible, while leveraging state of the art technology.
However, Fortune 1000s often perceive the value proposition of Causal AI a bit differently.
In summary, those are:
- Scaling Causal AI across the organization
- Actionable insights – Going beyond Predictions
- Explainability and Trust
- Human-Machine Collaboration
- Modeling and intervening on physical systems
This piece will take you through these 5 different value propositions, through the eyes of 5 different causaLens customers.
You can find individual case studies here.
With decisionOS by causaLens, enterprises can build & deploy decision making workflows, powered by Causal AI, to:
- Learn from the past: map cause-effect relationships of their KPIs and unearth the root causes of inefficiencies
- Make better decisions today: simulate different interventions (what-if scenarios) and design optimal next best actions (interventions)
- Move forward with confidence: productise, monitor and measure the impact of their decision workflows
Scaling Causal AI across the organization
Client profile: Fortune 10 global enterprise
Challenge: The client was ready to scale the use of Causal AI across its data science teams but lacked a tool to do so.
There are a growing number of open-source tools in the causality ecosystem. However they pose several challenges:
- Most of these packages focus on a single aspect of the causal modeling workflow and do not offer an end to end solution. This means a significant amount of effort is required to ensure underlying packages work well together.
- There are the usual risks associated with open source software, such as a lack of production system reliability or complex licensing arrangements.
- Developing capabilities based upon the open source meant the client would have to build, test, and refine interfaces to cover the full causal workflow, increasing time to value and defocusing their data science team from valuable and exciting modeling work.
Solution: decisionOS brings the entire Causal AI ecosystem together, allowing data science teams to experiment, build and deploy causal models in a single platform. decisionOS also enabled them to go beyond just causal models – building, deploying & measuring the impact of entire decision workflows, powered by the causal models, by leveraging decisionOps.
decisionOS was built for Data Scientists by data scientists. It provides the client access to the most complete library of causal discovery methods, causal model building architectures and decision intelligence engines.
Read more on build vs buy here.
Actionable insights – Going beyond Predictions

Client: Fortune 100 Financial Services Enterprise
Challenge: The client had accurate and robust ML models deployed to understand which clients were most likely to churn.
Unfortunately, while these models identified likely churners, they weren’t able to provide actionable recommendations.
This meant the client was misallocating its marketing budget, by running similar campaigns to all likely churners.

Solution: Leveraging decisionOS, the client was able to identify the causal drives of churn, identify likely churners and understand how different touchpoints were driving retention.
Leveraging decisionOS’ algorithmic recourse engine, they were able to create optimized and personalized marketing strategies maximizing the ROI from their retention campaigns.
decisionOps enabled them to productise, monitor & measure the impact of those strategies.
This new approach led to an improvement in retention of 17% without an increase in marketing spend.
Read more on the associated case study
Explainability and Trust

Client: Fortune 50 tech conglomerate
Challenge: The client’s data science team, various consultancies and a wide range of vendors had been working to develop countless ML models to solve a variety of business challenges.
However, there was a lack of clarity on how different model’s inputs impacted results and not able to answer “why”, leading to a lack of trust from business stakeholders.
Commonly used explainability methods like SHAP or LIME were trying to explain black box models only after the prediction is made (post-hoc) and use proxy models to do that – such approaches struggled to gain the trust of decision makers.
Read more on why current Explainable AI (XAI) doesn’t explain enough.
Solution: Unable to produce trusted models effectively, the client’s data science team turned to causaLens.
Leveraging decisionOS, they built robust causal models that did not sacrifice performance for explainability by leveraging our causalNet model.
causalNet is helping data scientists discover structural causal models that:
- Follow the cause-effect relationships of a given causal graph
- Can be constrained to discover simpler/linear relationships between variables or more complex, non-linear ones. The learned relationships can be easily visualised and inspected
- Can intuitively answer “why” they produce their predictions
- Accurately compute interventions & counterfactuals
Furthermore, using decisionOS’ Human-Guided Causal Discovery framework, they could incorporate the business team’s knowledge into such models, further increasing their confidence in them.
Through user friendly decisionApps, the business users were empowered to interact and interrogate the models driving trust and adoption.
Read more on the associated case study
Human-Machine Collaboration
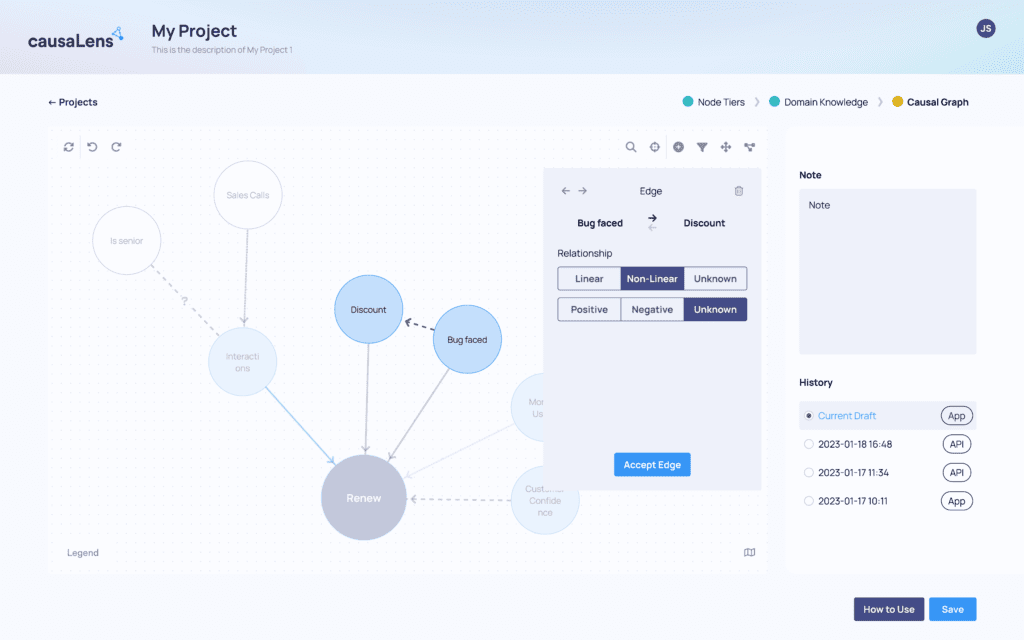
Client: $20bn Manufacturing Leader
Challenge: Leveraging their existing toolkit and expertise, the client had the ability to build two broad types of models:
- Knowledge based models, where domain experts determine the full model structure, making them more explainable and robust but limiting the complexity of the relationships that can be learnt
- Machine learning models, where the model parameters are learnt from the data, allowing for very complex relationships to be learnt but ignoring the underlying rules of the system
The client wanted the ability to build models that combined the best of data driven approaches and domain expertise.
Solution: decisionOS’ Human-Guided Causal Discovery allows domain experts to collaborate with data scientists and collect their knowledge through an easy to use interface.
Through this combination of domain expertise and algorithmic causal discovery, the client can create causal graphs in settings where data is limited, highly correlated or too high dimensional for purely algorithmic approaches to work.
At the same time, they managed to leverage the data to capture more complex relationships that the domain expert might have been unaware of.
The result is Causal Models that bridge the gap between knowledge based models and data-driven machine learning, capturing the best of both worlds.
Read more on the associated case study
Modeling and intervening on physical systems

Client: Fortune 100 technology conglomerate
Challenge: The client was looking to model the behavior of a physical system. The use-case was high risk and therefore required an explainable and generalizable model to get buy-in from the subject matter experts.
In their search for a solution, the customer explored the realm of traditional machine learning models, having previously worked with 6 different vendors and trying an in-house solution.
However, they encountered a significant roadblock when these models, which showed promise in controlled lab environments, faltered when exposed to the real world.
Traditional Machine Learning approaches, including deep learning, struggled to discover the structural, physics-based, relationships between variables in data. Instead, they were simply capturing statistical patterns (correlations) that didn’t follow any physical relationships. As a result, those approaches failed to generalise outside the lab.
Solution: Using causaLens’ decisionOS platform, domain experts were empowered to embed their unique knowledge of the system, ensuring the causal model respected the physics behind the system. Following the domain experts’ input, the causal discovery algorithms available in decisionOS managed to discover the complex, structural, relationships in data.
Furthermore, decisionOS allowed them to go beyond identifying faulty products, automatically identifying the root cause of issues with our root cause analysis capabilities.
decisionOS allows the customer to significantly shorten the costly R&D cycle, with fewer tests that brings better and much more trustworthy results.
Read more on the associated case study