Human-Guided Causal Discovery
Overview
Human-Guided Causal Discovery
Capturing cause-effect relationships in enterprise data is an essential step to trusted decision making – but it’s complex and requires the best of both algorithmic and human knowledge approaches.
decisionOS includes tools for seamless synthesis of both approaches allowing users to create their own personalized workflows powered by the most complete library of algorithmic causal discovery approaches.
Human-guided causal discovery early in the data science pipeline allows data scientists, business executives & regulators to align on the fundamental mechanics of a model’s decision making process, accelerating the development lifecycle & adding the necessary trust in the data science process
Collecting domain knowledge
The Human Guided Causal Discovery user interface allows for domain expertise to be captured in two broad ways:
- Tiers and superstructures where domain experts define which sets of variables can drive each other.
- Direct causal relationships where domain experts define specific relationships they know exist as well as prohibit those that are not possible.
This way we can collect knowledge that may not be present or is too difficult to determine from the data
Algorithmic causal discovery
Knowledge collected through the UI and use it to constrain the algorithmic causal discovery.
decisionOS provides data scientists with the most complete library of causal discovery algorithms suitable for different types of data and problems. These can all incorporate domain knowledge.
Domain expertise not only often improves the results of causal discovery, but also decreases the time to obtain results and allows for larger graphs to be discovered by reducing the search space.
Leverage LLMs’ Qualitative Knowledge
Generative AI enhances the causal graph discovery by introducing a domain-knowledge assistant to the causal discovery process.
The generative model offers directional causality suggestions along with accompanying explanations. This provides the practitioners an initial starting point, which enhances productivity.
LLMs trained on enterprises’ internal data are particularly powerful in this process, summarising the domain expertise of the entire enterprise
Validation
Business stakeholders, domain experts and data scientists collaborate to finalize the causal graph.
This iterative process leads to more accurate graphs being discovered but more importantly increases the trust of the domain expert in the modeling process. Through their involvement in the modeling, they have gained an understanding of how the model will be making decisions.
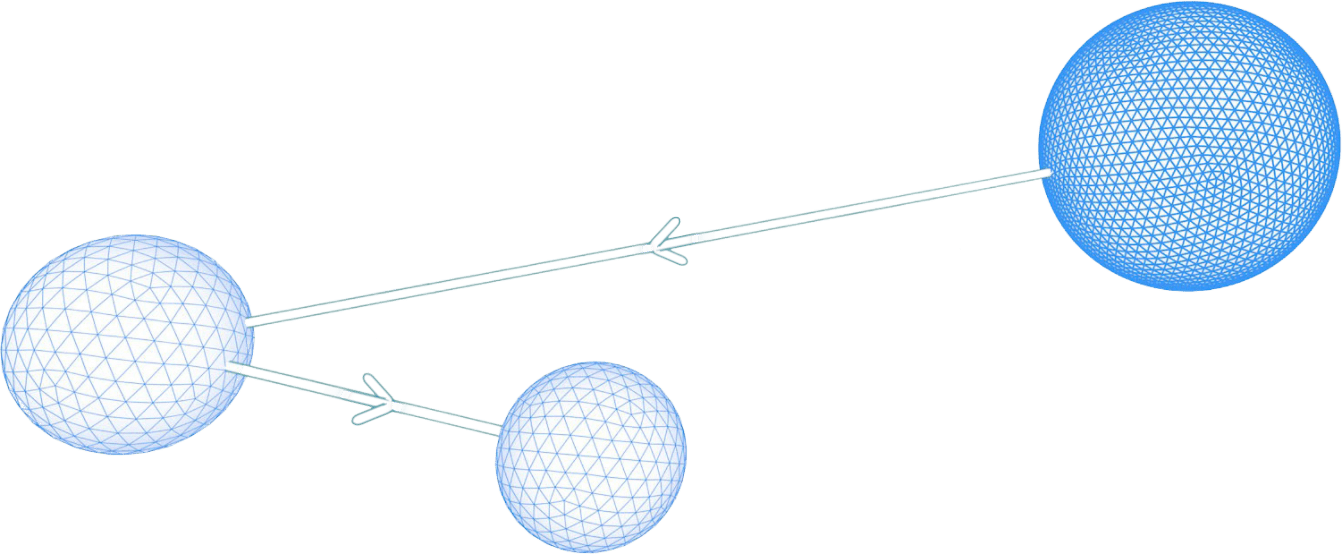