Causal AI & Gen AI Synergies
LLMs have made a noteworthy impact on the broader field of artificial intelligence. Nevertheless, they exhibit certain limitations when employed independently for enterprise decision-making due to issues related to consistency, transparency, and a deeper comprehension of causal relationships within data.
Read more on our blog “Enterprise decision making needs more than LLMs“.
Causal AI, by contrast, inherently offer a transparent framework for revealing these cause-and-effect relationships, building causal models and offering actionable recommendations that decision makers can trust.
We launched 3 core features where Gen AI & Causal AI collaborate to help enterprises accelerate the time to trusted, AI-powered decision workflows.
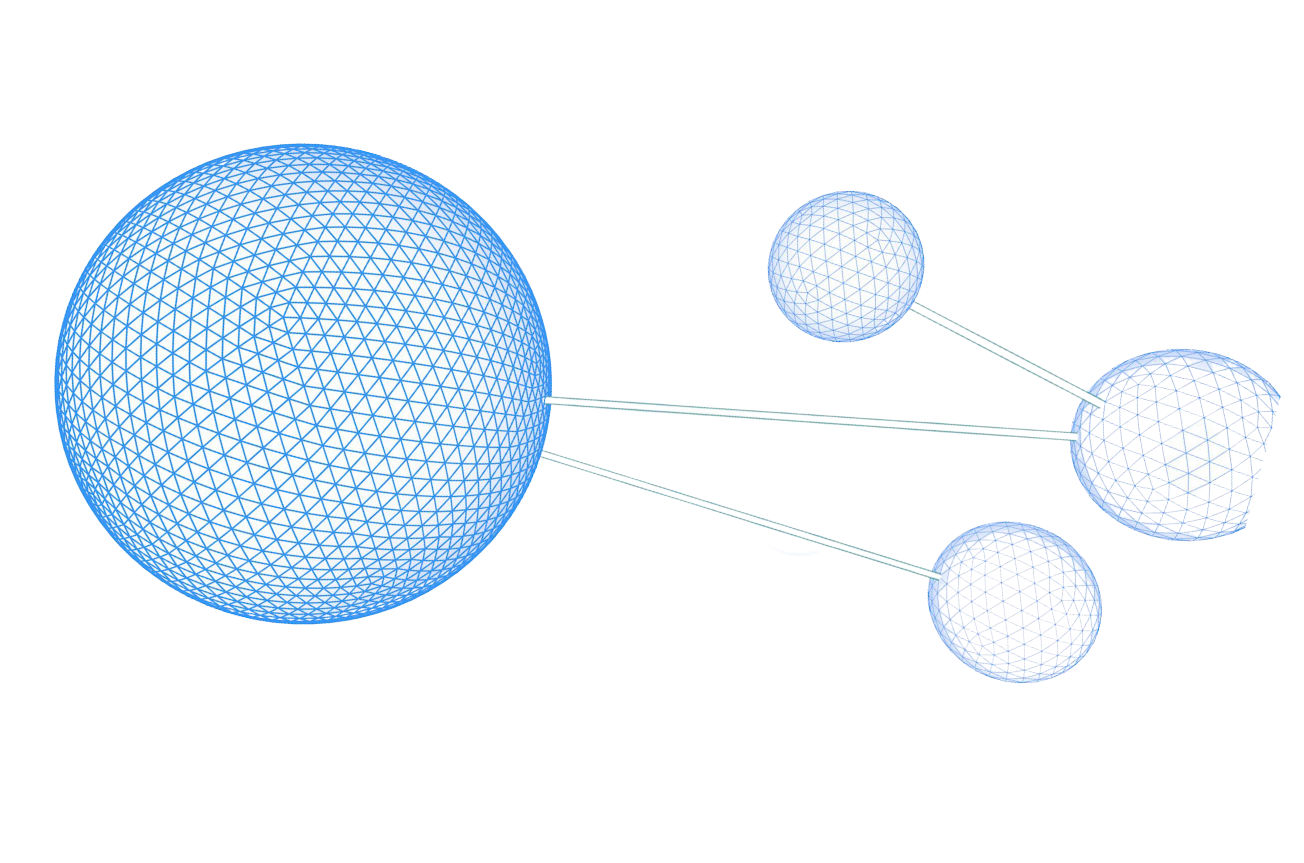
Embed Causal Thinking into LLMs
The causal diagram serves as a visual representation of causal relationships and the underlying data generation process. These encapsulate a deeper representation of knowledge compared to conventional predictive methods.
The integration of human-refined causal assets with large language models provides a synergy and creates responses distinct from those from a generative model in isolation. This offers the potential to enhance the quality of responses and address some of the trust-related challenges commonly associated with generative models.
For example, in the analysis of churn-contributing factors, the responses now contain causal drivers, as a representation of causal assets has been embedded within the LLM.
Build Better Causal Graphs
causaLens has pioneered human guided causal discovery, that blends domain expertise with the best algorthmic approaches in order to discover cause-effect relationships and causal graphs.
Generative AI enhances this solution by introducing a domain-knowledge assistant to the causal discovery process. The generative model offers directional causality suggestions along with accompanying explanations. This provides the practitioners an initial starting point, which enhances productivity.
It’s important to note that the human element remains integral in the loop, as users retain the ability to accept or reject these suggestions. Within the DecisionOS platform, this entire process is seamlessly integrated, delivering an enhanced and user-friendly experience
Explain Causal Relationships
Causal graphs inherently offer transparency, a quality appreciated by many causaLens customers for their effectiveness in communicating to diverse enterprise audiences.
Causal models embed the mathematical relationships that flow through the graph. A generative model can simplify the interpretation of the causal model in a language more accessible to business users.
This enhances the accessibility and understanding of these models. To consume and understand such models, a business analyst does not need to possess the skillset of a data scientist.