Research
Access the latest research in Causal AI development
Mayo Clinic x causaLens: Towards Causal Analysis of Genetic Factors for Colorectal Cancer
Mayo Clinic and causaLens researchers leveraged Causal AI techniques to discover causal drivers of Colorectal Cancer. In this paper, we […]
Read moreData Generating Process to Evaluate Causal Discovery Techniques for Time-Series Data
causaLens’ NeurIPS 2020 paper sets out a framework for benchmarking causal discovery techniques time-series data. causaLens researchers Andrew Lawrence, Marcus […]
Read moreDomain Knowledge in A*-Based Causal Discovery
Causal discovery has become a vital tool for scientists and practitioners wanting to discover causal relationships from observational data. While most previous approaches to causal discovery have implicitly assumed
Read moreUnsuitability of NOTEARS for Causal Graph Discovery
Many popular causal discovery algorithms have significant limitations in applied settings. causaLens’ Marcus Kaiser and Maksim Sipos show how one […]
Read moreAn Overview of the Methodologies of Causal Discovery
Until recently, discovering cause-and-effect relationships involved conducting a carefully controlled experiment or else relying on raw human intuition. Technological breakthroughs […]
Read moreEquality of Effort via Algorithmic Recourse
AI systems are increasingly used in many socially significant applications, such as loan approval, hiring decisions, legal processes, and healthcare, sometimes encoding existing human
Read moreCausal Analysis of the TOPCAT Trial: Spironolactone for Preserved Cardiac Function Heart Failure
Complex trials with heterogeneities in the data pose a significant challenge for traditional techniques of statistical analysis. Suboptimal patient cohort […]
Read moreA Causal Analysis of Harm
Defining harm is essential for dealing with the many legal and regulatory issues around the growing integration of autonomous systems in society. Consider, for example, the question of harm in accidents involving self-driving cars.
Read moreOn Testing for Discrimination Using Causal Models
causaLens’ own Hana Chockler in collaboration with Cornell’s Joe Halpern set out novel causal methods for detecting discrimination. The paper, […]
Read more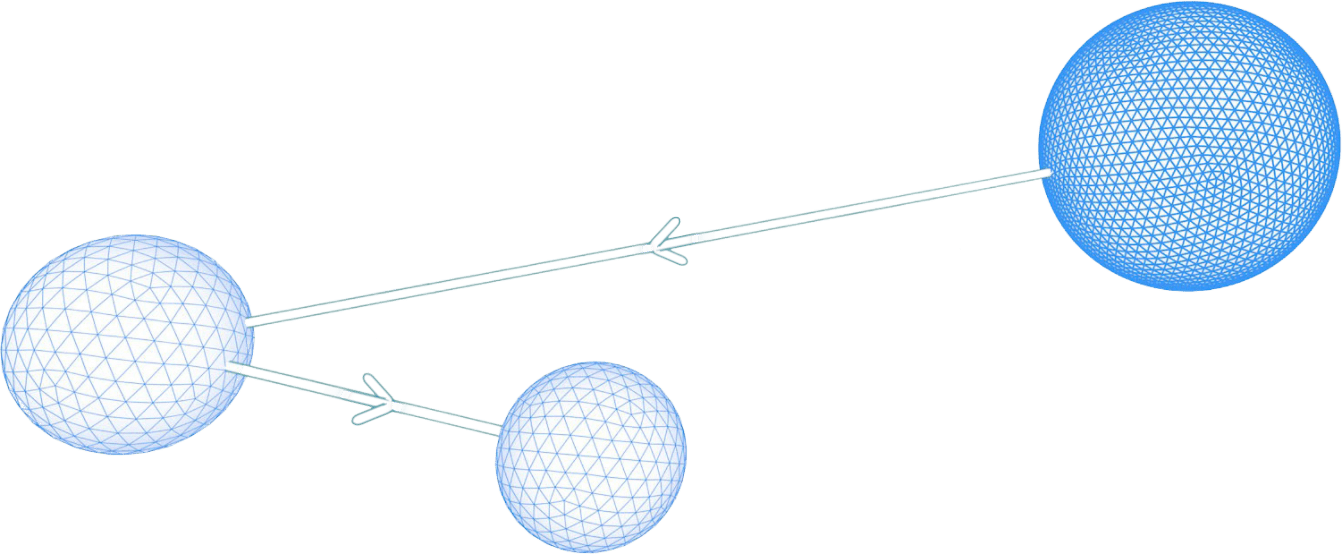