- Research Papers
Equality of Effort via Algorithmic Recourse
23 November 2022, 17:25 GMT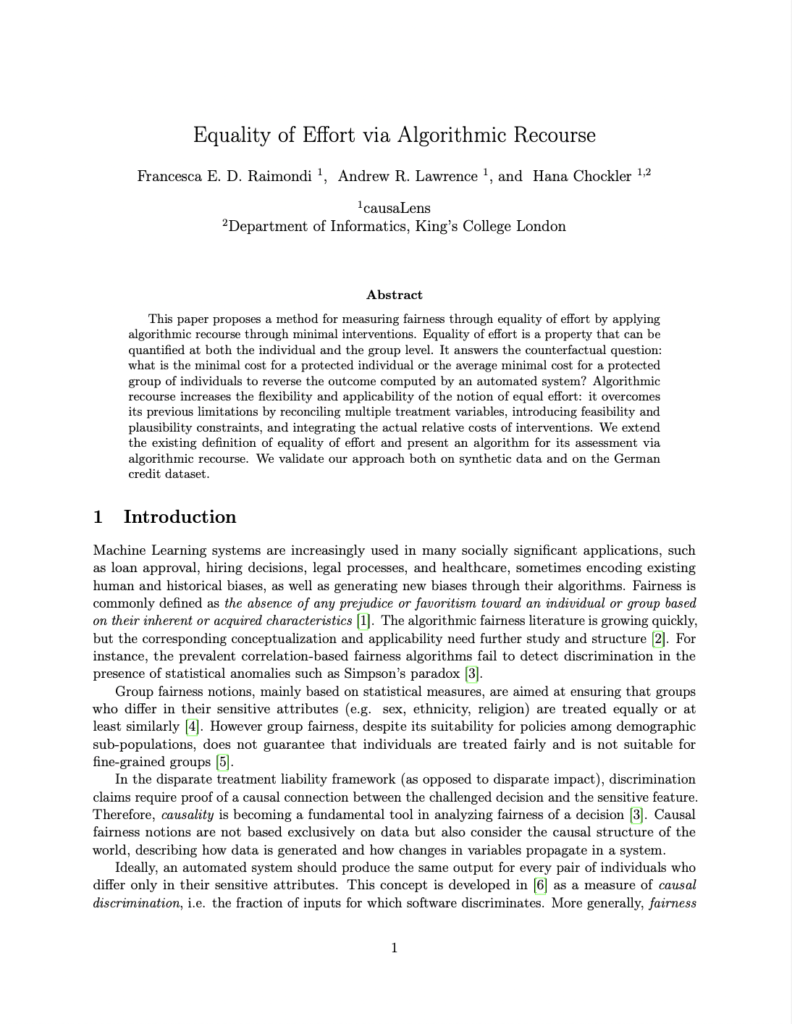
AI systems are increasingly used in many socially significant applications, such as loan approval, hiring decisions, legal processes, and healthcare, sometimes encoding existing human and historical biases, as well as generating new biases through their algorithms.
In the disparate treatment liability framework (as opposed to disparate impact), discrimination claims require proof of a causal connection between the challenged decision and the sensitive feature. Therefore, causality is becoming a fundamental tool in analyzing fairness of a decision.
The notion of equality of effort, based on similarity measures among individuals, can help answer counterfactual questions like by how much should an applicant’s credit score improve so that their loan application is approved?, and judge discrimination from the perspective of equal effort among groups.
Equality of effort is a property that can be quantified at both the individual and the group level. It answers the counterfactual question: what is the minimal cost for a protected individual or the average minimal cost for a protected group of individuals to reverse the outcome computed by an automated system?
Equality of effort establishes whether the effort to reach a certain outcome is the same for the protected and unprotected individuals and groups. A treatment variable is studied as to what change is required for an individual or group to achieve a certain outcome.
We propose a method for measuring fairness through equality of effort by applying algorithmic recourse through minimal interventions, i.e. the actions with minimal cost required for reversing an unfavorable decision.
This work, presented at the NeurIPS 2022 workshop Algorithmic Fairness through the Lens of Causality and Privacy, extends the existing definition of equality of effort and presents an algorithm for its assessment via algorithmic recourse, and validates this approach both on synthetic data and on the German credit dataset.