- Blog
From Models to Decisions
13 June 2023, 11:20 GMTAlgorithmic Recourse
Suppose you have constructed a causal model that is able to quantify how exercise, medication and stress management tools affect blood pressure – the natural question is: how to use this information to get the optimal intervention, for each individual? Each intervention (for instance, taking a daily medication) will carry its costs and risks – as well as an expected effect on blood pressure. Furthermore, combining different interventions can also have a nonlinear effect on the outcome.
Algorithmic recourse is a decision intelligence engine that allows us to, given a causal model, answer the above question in a seamless way. Effectively, it’s giving is “the set of interventions with the minimal cost that is able to achieve a certain goal”. This is a tool that can be used in any setting where there’s a decision boundary to be crossed: for blood pressure, this can be phrased as reaching 120/80 – for customer retention, it could be ensuring with 50% confidence that a customer will not churn.
Root Cause Analysis
Root Cause Analysis (RCA) is a systematic process employed to identify the fundamental causes underlying a specific problem or event. In the context of causal inference, RCA is primarily used to determine what factors or sequence of events led to the observed outcome. Unlike many causal inference techniques that might focus on correlations or associations between variables, RCA aims to delve deeper to uncover the true underlying causes, ensuring that remedies are targeted at these root causes rather than just addressing the symptoms.
Root Cause Analysis finds applications in various domains including healthcare, engineering, business, and information technology. For example, in healthcare, RCA might be used to investigate the cause of an increase in patient readmissions or to understand the underlying reasons for a sudden outbreak of infections in a hospital. In engineering, it can be applied to analyze the failure of machinery. Businesses might use RCA to investigate the causes behind a drop in sales or customer satisfaction. In information technology, RCA is often employed to ascertain the causes of software bugs or network failures.
The adoption of Root Cause Analysis comes with several benefits. Firstly, by identifying the underlying causes of a problem, RCA ensures that interventions and solutions are more effective since they address the core issues. This, in turn, prevents recurrence of the problem. Secondly, RCA often helps in optimizing resources, as it aids in focusing efforts and investments on areas that will have the greatest impact. Moreover, RCA fosters a culture of continuous improvement and learning within organizations, as it encourages a thorough understanding of processes and systems.
Despite its merits, Root Cause Analysis has its own set of limitations. Firstly, RCA can be a time-consuming process, especially in complex systems with numerous interrelated factors. Additionally, there is a risk of bias in the analysis, as individuals conducting the RCA might have preconceived notions about the causes, which can affect the objectivity of the investigation. Moreover, in certain instances, the root cause might be influenced by factors that are difficult or impossible to measure, and RCA might not always provide a complete picture in such scenarios.
In decisionOS, we have implemented two proprietary techniques to tackle the problem: Interventional and counterfactual RCA:
- Interventional RCA attempts to find out which variable of an event needs to be fixed in order to change the unfavorable outcome of a target to a favorable outcome. In doing so, this method intervenes on every potential root cause, thereby changing the value to a control value, given by a mean, median, user-provided value, or a user-defined callable.
- Counterfactual RCA attempts to find out which variable of an event, if its value had not been an outlier, changes the unfavorable outcome of a target to a favorable outcome. In doing so, this method computes a counterfactual prediction of the target variable for every potential root cause. While the factual data is the actual value of a potential root cause, the counterfactual value is given by the control value, given by a mean, median, user-provided value, or a user-defined callable.
In summary, Root Cause Analysis is an indispensable instrument in the toolkit of causal inference, acting as a beacon that guides stakeholders through the labyrinth of events and factors to discover the true culprits behind problems. With its focus on the underlying causes, RCA is a necessary condition for crafting stable solutions and allowing practitioners to proactively act to mitigate potential problems and optimize their outcomes.
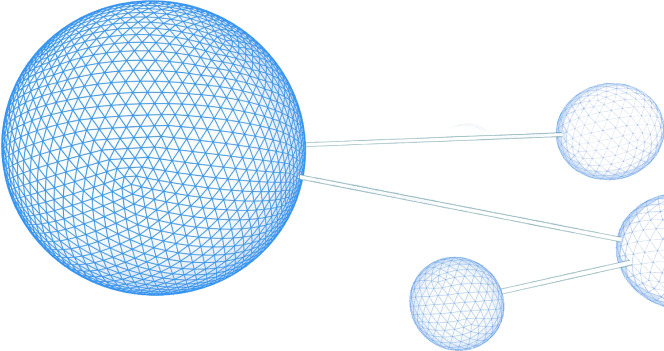