Events
Learn about the latest events and where we will be showcasing our latest developments.
Big Data & AI Toronto
This event has been providing a unique platform for IT decision-makers and data innovators. Big Data and AI Toronto allows them to explore and discuss insights, showcase the latest innovative projects, and connect with other data and analytics professionals
Read moreFX Markets Europe 2022
Bringing together risk management and risk transfer professionals from across the capital markets industry, Risk Live combines four events under […]
Read moreActual Causality, Explanations, and Fairness
Dr. Hana Chockler introduces the theory of actual causality as defined by Halpern and Pearl. This theory turns out to be extremely useful in various areas of computer science due to a good match between the results it produces and our intuition.
Read moreBig Data LDN
Big Data LDN (London) is the UK’s leading free to attend data & analytics conference and exhibition, hosting leading data and analytics experts, ready to arm you with the tools to deliver your most effective data-driven strategy.
Read moreAI & Big Data Expo
Topics covered include Business Intelligence, Deep Learning, Machine Learning, AI Algorithms, Data & Analytics, Virtual Assistants & Chatbots
Read moreQuantMinds – Why Causal AI Prevents Overfitting
The current state of the art in machine learning relies on past patterns and correlations to make predictions of the future.
Read moreAn Intro to Causal AI for Asset Management
causaLens' Ben Steiner will be speaking on Causal AI and its uses in asset management.
Read moreAI in Finance Summit
A financial industry event to discover cutting-edge advancements in AI & Machine Learning and their adoption in financial services to increase efficiency & solve challenges.
Read moreActual causality, responsibility, explanations, and fairness – a bird’s eye view
causaLens' own Hana Chockler speaks on her leading research on actual causality, and its relationship to core concepts such as responsibility, explanation, and fairness.
Read more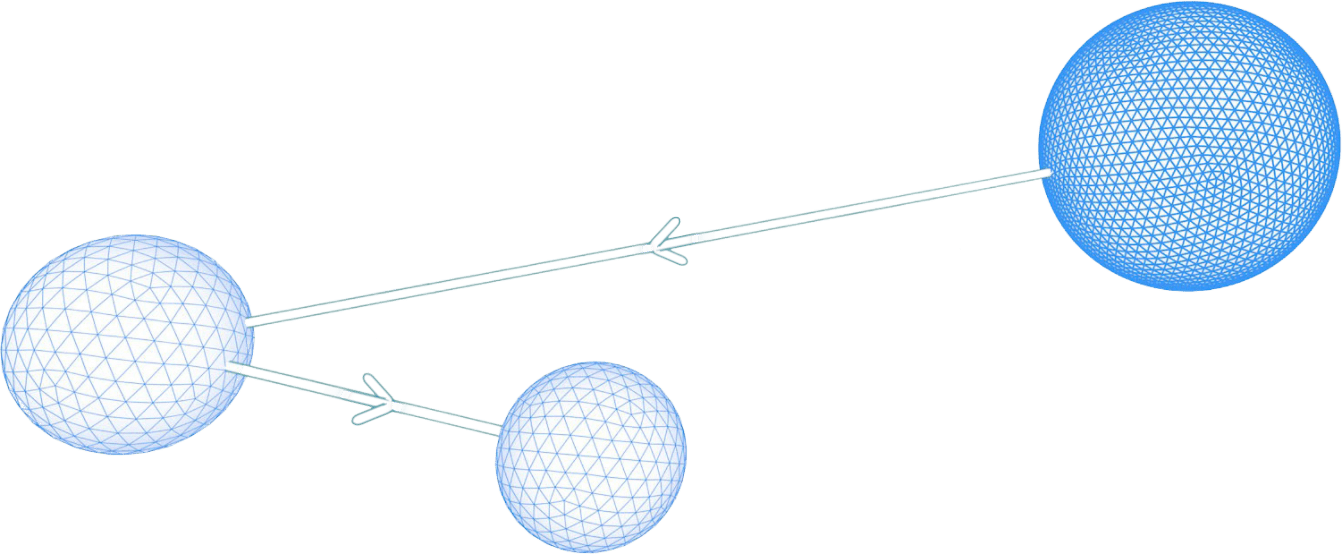