Why data providers need Causal AI
At a glance
- To compete in a crowded market, data providers must be able to move up the data value
chain, delivering not just raw data but signals and solutions. - Conventional data science approaches and analytics platforms fail to identify signals in
noisy financial data. - Causal AI separates out causal signals from spurious correlations. Leading data vendors
and stock exchanges use Causal AI to augment their offerings and win market share.
Challenges for data providers
Prove data has value
Data vendors must be able to prove their data has value for potential customers to win business. When purchasing financial data, a top concern for 50+% of customers is the difficulty of identifying value in big data.
Vendors need to provide impactful use cases — something 60+% of data vendors offer today, as compared to just 10% five years ago.
Vendors also need to demonstrate their data has marginal value, over and above existing datasets and products. Most datasets appear valuable in isolation. However, many datasets are highly correlated, expressing similar facts about the world.
Provide value-added solutions
Alpha-generating solutions are becoming table stakes for data vendors. Vendors need to leverage the best tech to discover market inefficiencies and risk premia. Insights need to be unique and stable. Customers place a premium on early identification of regime shifts and emerging trends. Vendors must be able to handle “low-signal-to- noise” time-series data, where insights are buried deeply and surrounded by lots of noise.

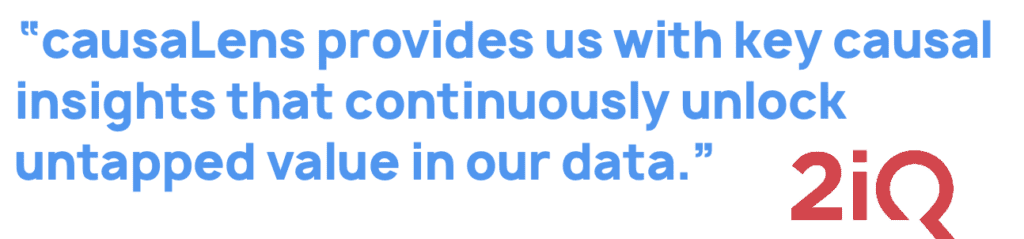
Cater for diverse customer needs
Different customer segments vary in their degree of quantitative sophistication, and have very different data needs.
Systematic traders need co-location of data and analytics capabilities. Traditional quant teams expect curated signals. Traditional investment teams on both the sell-side and buy-side need explainable insight-as-a-service. FinTech and smaller players need low-maintenance advanced analytics platforms and flexible access to data science talent. Providers need to market and productize for these diverse customer needs.
Product innovation and pricing optimization
Vendors need to understand sources of value in their data to maximize revenue. This includes hybridizing datasets to create new revenue streams and setting sophisticated pricing strategies that reflect the value-generating potential of different data assets.
Conventional machine learning & standard predictive analytics platforms leak value
Manual data science is slow, expensive and repetitious.
Using the same tech on the same data crowds out value.
Conventional ML overfits to spurious correlations in big, noisy financial datasets. This renders the “signals” and “solutions” they “discover” valueless to real-world users.
Conventional ML is a black box: insights lack explainability and trustworthiness.
Conventional ML crashes when confronted with new or unexpected scenarios, making it fragile in deployment and poorly suited to many high-value use cases.
Benefits of Causal AI solutions
Identify orthogonal signals
Causal technology can determine how a new dataset interacts with existing datasets and decide whether a new variable is a true causal driver of a given target, enabling you to identify true sources of value in your data assets.
Discover value as the world changes
Causal AI adapts far quicker than conventional technologies to disruptive events and new economic regimes, due to low reliance on spurious correlations and modelling of the “data-generating process”. It generates reliable early warning signals, macroeconomic forecasts and continuous intelligence in a dynamic world.
Extract intelligence from “low signal-to-noise” data
causaLens’ platform is the only one on the market that autonomously discovers insights in low signal-to-noise ratio time-series data, that are ubiquitous in finance.
Eliminate black boxes
Causal AI products extract signals and combine datasets in a way that is completely explainable to you and your customers.
Automate pipelines
causaLens’ platform automates the most sophisticated Causal AI algorithms. It autonomously converts raw, unclean data into signals with proven value. We perform 1000s of research iterations to maximize the chance of finding a new data product.
Turbocharge signal discovery
The automated Causal AI platform dramatically reduces time-to-new-product and speeds up signal discovery by 10x.
Operationalize your data products
We can make sure your new data products run smoothly — from satisfying SLA agreements to resolving missing data issues.
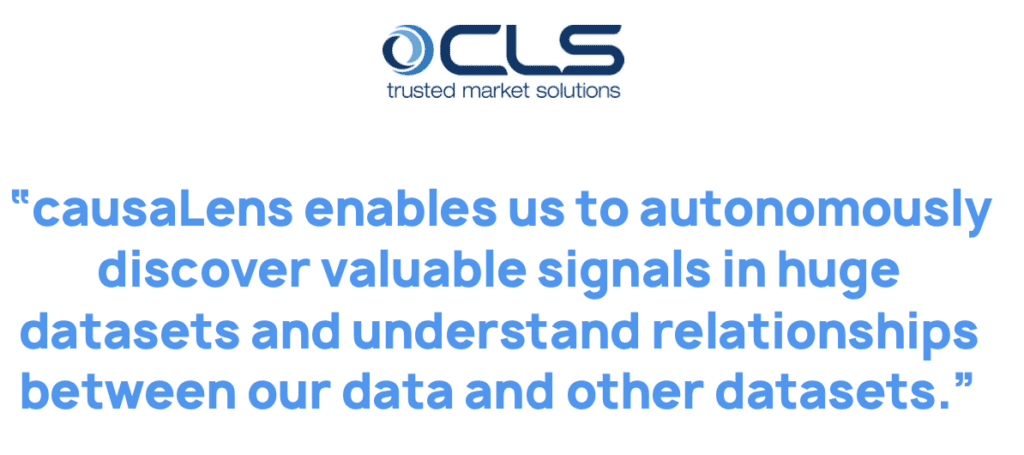