Customer Case Study: Manufacturing Root Cause Analysis
$15M annual value unlocked through reduced downtime for a leading commodity manufacturer
$20bn Commodity Manufacturer
Manufacturing
Root Cause Analysis
Reduced downtime yields $15mn value
The Challenge
A leading commodity manufacturer came to causaLens to help optimize their Overall Equipment Effectiveness (OEE) at one of their particularly problematic manufacturing plants. They faced instability issues with some of their critical machines – often 10s of faults per week – leading to significant downtime and reduced production efficiency.
Identifying the root cause of the problem through physical inspections was challenging due to the machine’s large size and complexity. When a fault occurred, significant effort went into troubleshooting the fault by the subject matter experts.
The client required a solution to identify why the faults occurred and also wanted to learn optimal settings for the machine to prevent future issues.
-
1Losses in revenue due to reduced OEE
Quickly identifying and resolving faults as they occur is critical to maximizing the OEE of the plant.
-
2Manual processes are time-consuming
Machine size and complexity make physical inspections challenging and inconclusive while drawing significant subject matter expert resources.
-
3Existing ML can make things worse
Correlation-based ML approaches can’t identify true root causes, leading to misdiagnosed faults and prolonged resolution times.
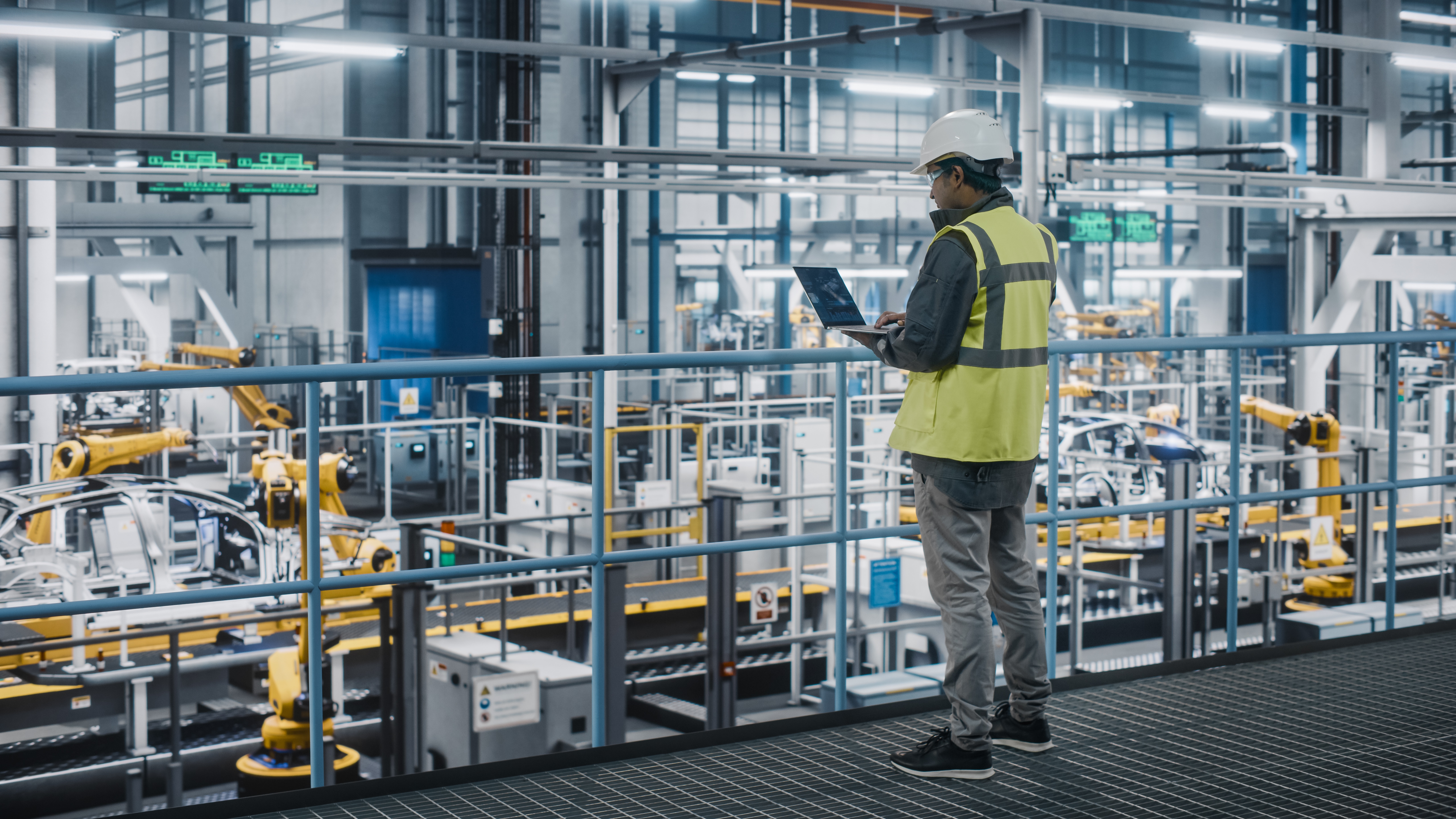
The Solution
causaLens collaborated with the manufacturer on the implementation of decisionOS, our Causal AI platform, to learn the machine dynamics and understand the relationships between physical measurements, such as temperature pressure, and their impact on machine stability. This can then be used to identify root causes when faults occur quickly.
- We developed a Time Series Causal Model, describing the manufacturing pipeline as a dynamic system.
- The solution leveraged the causaLens Root Cause Analysis Engine to identify the root causes of historical instabilities.
- Causal insights enabled on-site engineers to correct previously unseen faults, improving production efficiency.
Results and Benefits
causaLens’ solution has contributed to a value of over $15 million through reduced production downtime. The client is leveraging the decisionOS Root Cause Analysis (RCA) engine to identify faults within the machines.
The solution’s success in uncovering root causes allows for the identification of optimal settings to minimize instabilities while maximizing production rates. The client has made lasting changes to the machine to reduce the number of faults that occur, and decisionOS is also used on an ongoing basis to identify new faults quickly. The time from instability onset to faulty component identification has also been reduced.
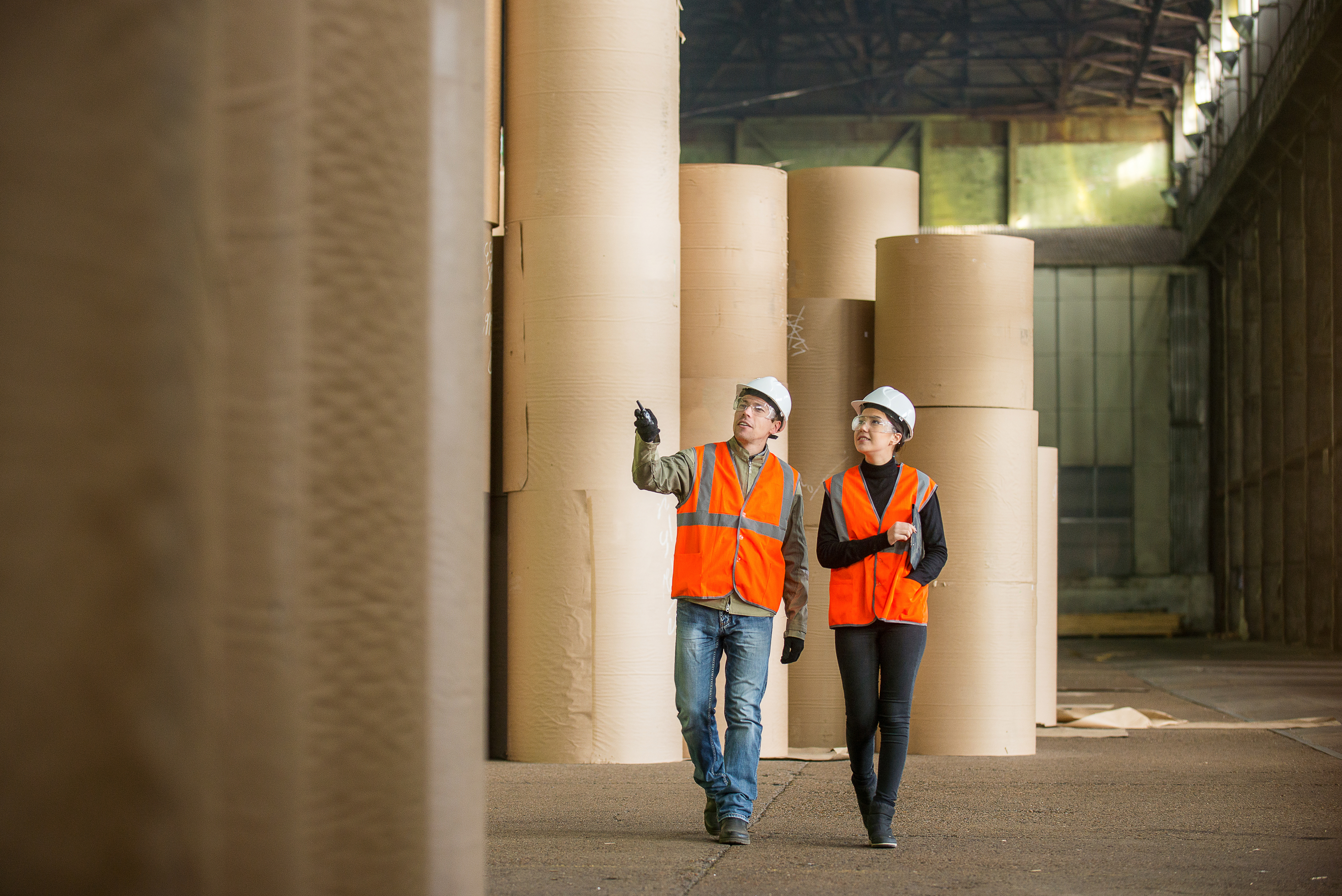