Customer Case Study: Deposit Modeling
A large European bank estimates return of €12mn using decisionOS to better understand and manage deposit risk
A leading European retail and commercial bank
Banking
Deposit Behavioral Modeling under Changing Macro Conditions
Yearly Return €12M on investment
The Challenge
A large European bank’s Treasury and Asset and Liability Management team had long wanted a better way of understanding and planning around changes in deposit levels to inform risk management and PnL strategies. There was also increasing demand from regulators to better explain risk management decisions given the changing external economic environment due to the pandemic, money supply, inflation and interest rates changes.
The Bank’s existing approaches were predominantly trend based and could not factor in how the changing conditions would affect future balance levels. While more complex modeling techniques had been tried – they had ended up as black boxes that could not be trusted or explained to regulators, and they had also been unstable in the way they assigned weights to input features.
-
1Explainability
Regulators demand trustworthy and explainable solutions
-
2Learning from correlations
Trend based approaches proved to be unstable
-
3Simulating scenarios
Pressure from regulators to explain risk management decisions in changing economic environments
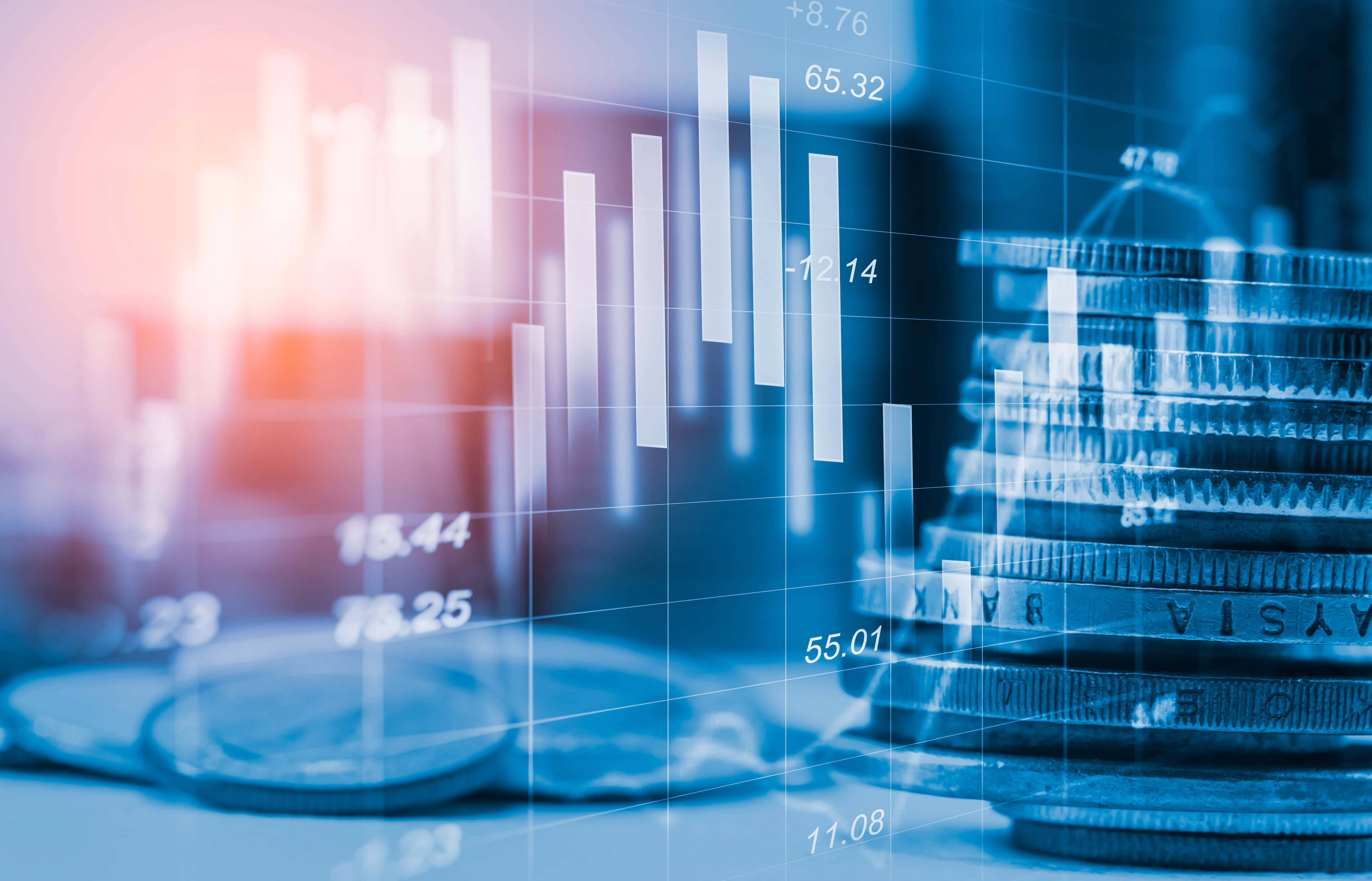
The solution
The Bank partnered with causaLens to provide technology to help the banks analytics teams to derive the underlying causal structure driving changes in deposits levels. Using decisionOS the bank was able to:
- Encode economic domain knowledge while also leveraging algorithmic causal discovery
- Build a causal model capable of running interventional and counterfactual modeling to simulate the effects of different scenarios on deposit levels
- Build a decisionApp, allowing business users to get intuitive explanations for historic balance movements and leverage the full power of counterfactuals to forecast future balance levels under different scenarios
Results and Benefits
The tests showed that the model could accurately explain the key drivers of deposit level changes and the strength of these effects. As a result the bank was able to more finely manage hedging strategies and LCR levels leading to increased profitability via cost reductions and increased NII (net interest income). The overall financial benefits were estimated to be in excess of 12M Euros per annum.
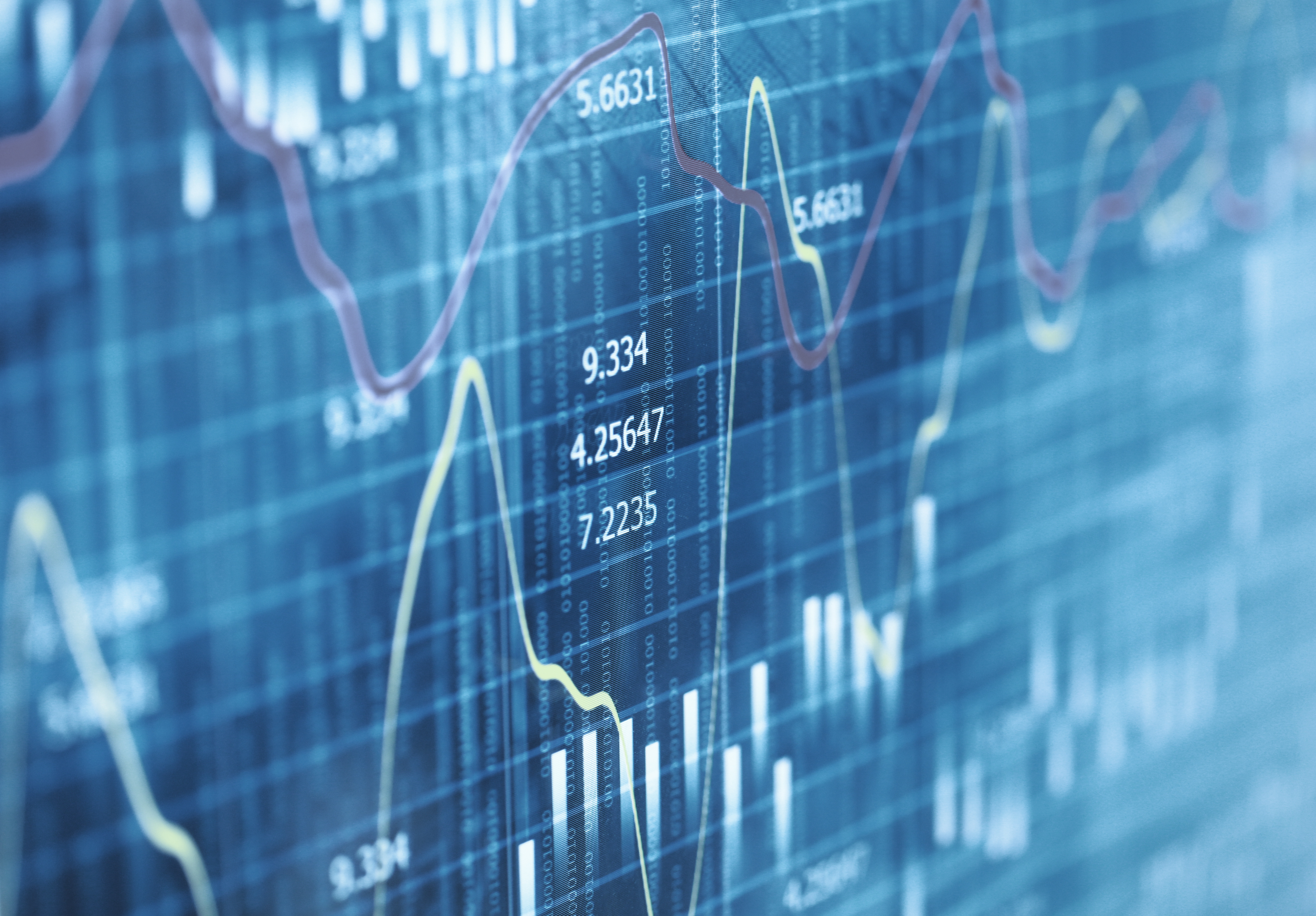