Decision Optimization
Overview
Beyond predicting. Act to change outcomes.
Our Decision Optimization packages determine the actions required to achieve your desired outcome, while respecting your business constraints. It allows you to generate actionable recommendations to solve your business challenges:
- The ability to generate accurate and actionable recommendations with recourse is only possible with causality.
- Embed business constraints directly into the optimization engines to ensure outcomes respect the real world.
- Act with confidence knowing why actions were suggested with built-in explainability tooling to enhance trusted decision making.
Cause and effect guides the best course of action.
Decision Optimization is only possible with an understanding of cause and effect relationships. This is necessary to quantify how certain actions would influence other variables.
Thanks to our optimizers you don’t need to worry about the complexity of searching to identify the best set of actions, this is done automatically for you.
Ground actions in the real world.
Configure seamlessly the decision optimization engines to respect the real world constraints which impact your business challenge:
- Actionable variables: Which variables can be acted upon in the real world and how they can change. For example the amount of discount a customer receives is actionable, while their age is not.
- Action cost: Define relative costs of performing actions, to allow the recourse engine to weigh up multiple options.
- Cost function: Select from pre-populated cost functions, or provide your own, to guide the optimization along the correct path.
Know why actions are recommended.
Use unique built-in explainability tooling to understand both individual and group-level results. Decision Optimization explanations provide:
- Plain text explanations of why recommendations were selected, allowing non-technical users to gain confidence in actions.
- How different recommendations compare with one another, and their relative costs.
- The effect the proposed actions would have on the other variables.
cai-optimization
The cai-optimization package aims at finding optimal interventions in structural causal models to maximize custom objective functions.
This flexible framework allows for solving many problems, from maximising a business KPI, to finding best actions under a budget, to designing better experiments.
algorithmic-recourse
The algorithmic-recourse package aims at identifying the interventions required to produce a given outcome.
Under the hood, the package discovers the actions required for reversing unfavourable decisions across a range of counterfactual scenarios e.g what is the most cost-effective way to retain a customer who is predicted to churn
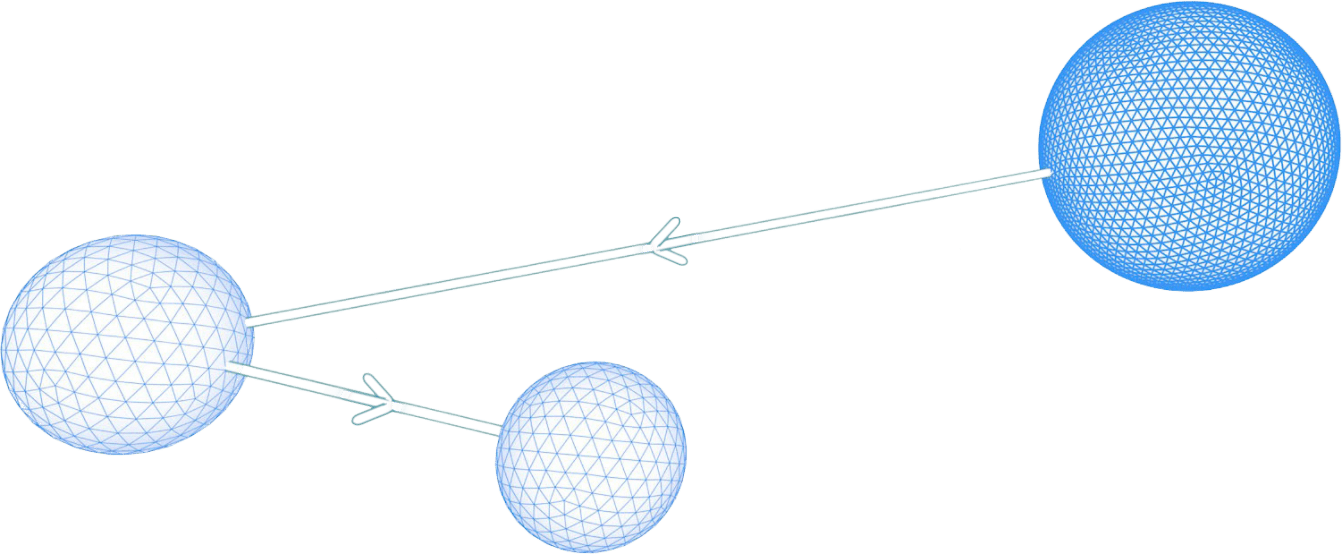