Causal Fairness
Don’t settle for less: Deliver fair and unbiased results.
As algorithmic decision making becomes increasingly prevalent it is critical that model inputs and outputs are carefully monitored to prevent discrimination, and de-risk decision making.
decisionOS provides a range of cutting edge capabilities to mitigate bias:
- Fairness requires causality: Correlation-based fairness metrics fail to correctly capture real world nuances.
- Fairness decision algorithm: Selecting the correct fairness metric can be overwhelming with conflicting options which vary from use case to use case. decisionOS provides a simple fairness decision algorithm to guide your selection.
- Fairness engine: Ingest a trained causal or non-causal model and generate a suite of fairness metrics to rapidly assess bias.
Mitigate bias with causality.
Correlation-based fairness metrics fail to detect discrimination in the presence of statistical anomalies such as Simpson’s paradox. Furthermore, disparate treatment claims require proof of a causal connection between the challenged decision and the sensitive feature. Therefore fairness requires causality.
decisionOS provides an extensive selection of causal fairness metrics.
Find the right fairness metric.
A universal definition of fairness does not exist, and so there exist many, many metrics for analyzing fairness. Selecting the best metric for your use case can be a complex and overwhelming task. Furthermore, using an inappropriate fairness metric can result in misleading results.
To counter these challenges, decisionOS uses a fairness decision algorithm which helps you to select from the 18 fairness metrics provided and find the best fit for your use case.
Place bias under the microscope.
The fairness engine ingests a trained machine learning model, causal or correlational, and based upon your configuration automatically allows calculation of a large catalogue of different fairness metrics.
The result is a wealth of information allowing you to find and rectify deep-rooted biases within your model and datasets.
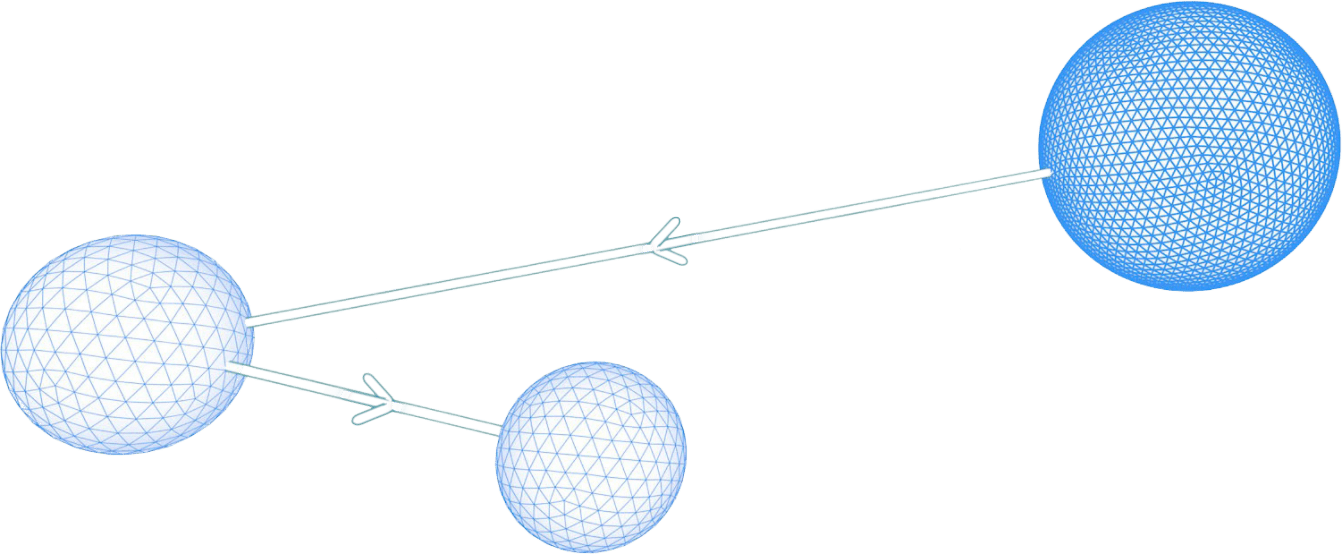