How to Connect Business Teams and Data Scientists
Increase the relevance of data science to the business!
Causal AI models are premised on business insight, and directly enhance decision-making. Data science teams that leverage Causal AI enjoy radically increased influence on the enterprise.
Many clients come to us with sophisticated AI projects that are failing to make an impact. They aren’t alone. 60% of investment in AI is wasted because analysis and insights are not tied directly to business decision-making, and key decision-makers ignore data science results 78% of the time.
There’s a disconnect between business decision-making and data science projects, and it’s resulting in missed opportunities and wasted investment. What’s going wrong? And how can enterprises effectively connect business stakeholders and data science teams?
Why is there a disconnect between businesses and data science?
Reason 1. Business knowledge and constraints aren’t integrated into models
In all likelihood, your enterprise’s data scientists are building models without meaningful input from the business. Stakeholders may tell data scientists to include or exclude certain variables. But that’s the extent of their guidance. The way features interact with each other, their relationship to the target variable, the broader business context, budgetary constraints—all that nuance is left on the table.
Note that this is not a failing of either your data scientists or business stakeholders—it’s a limitation of current enterprise technology.
The upshot is data science projects are often heavily delayed or fail outright (in approximately 90 percent of cases, according to a range of analyses).
Business stakeholders find that models built without their inputs make false or nonsensical assumptions. Attempts to sanity-check, stress-test, and improve models in light of the business’ insights result in lengthy delays as data scientists scramble to respond to feedback. Iteration cycles between data and business teams repeat multiple times.
Reason 2. Data scientists build predictive models, but predictions are a small aspect of business decision-making.
Enterprises have to make many critical decisions: what to sell, at what price, to which customers, with which marketing campaigns, fulfilled via which supply chain. Each nontrivial enterprise decision requires lots of inputs that may include strategic context, budgetary constraints, logistical constraints, commercial intuition gathered from a wide range of stakeholders, A/B testing or randomized controlled trials, and trial-and-error experimentation.
Whereas machine learning algorithms just make predictions, or they classify observations into groups—and predictions and classifications only make up a tiny fraction of the input needed for business decisions. As a result, machine learning systems often end up as solutions in search of business problems.

Lengthy iteration cycles and high failure rates are typical of enterprise data science projects.
Two keys to connect business and data science teams
Key 1. Don’t ask what your data scientists can do for you, ask what you can do for your data scientists
Causal AI reimagines the traditional data science playbook, uniting business stakeholders, data scientists, and machine intelligence in the model-building process.
In essence, the business user formulates a hypothesis, which the machine, led by the data science team, then investigates and refines.
Let’s take a look at how this works. We’ll use a customer retention model as an example.
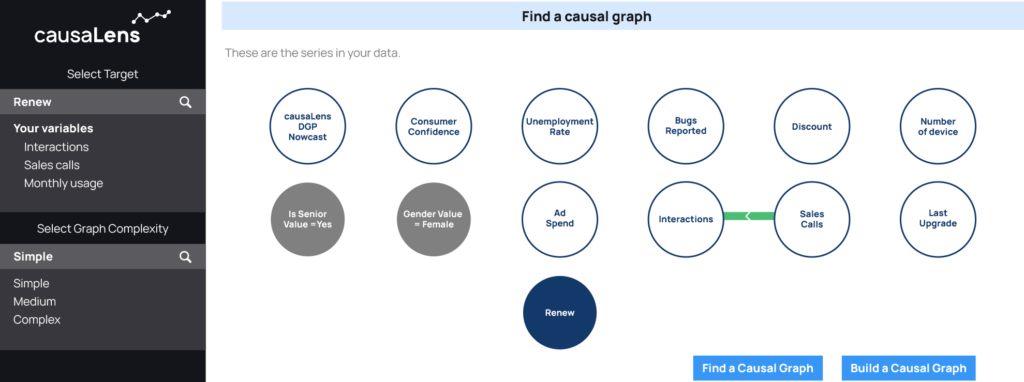
The business stakeholder starts by defining the target—namely the probability of renewal—and selecting any relevant features. How does ad spend impact renewals? What about macroeconomic factors like the unemployment rate? The business user can drag and orient arrows between features to create a “napkin model” of the causal dynamics
of renewal.
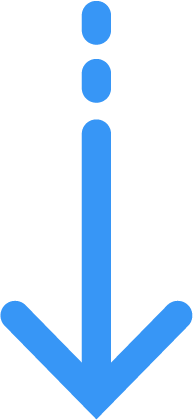
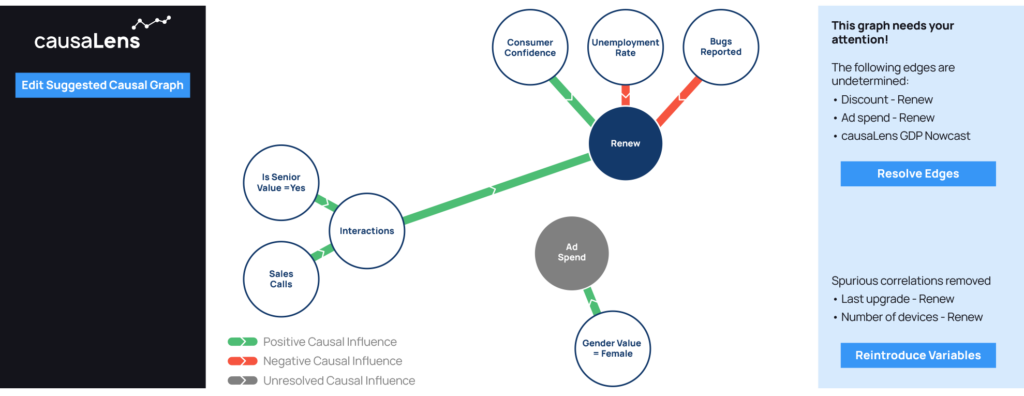
The baton is then handed over to the machine, led by data scientists, which runs experiments using powerful causal discovery algorithms to verify the business user’s hypothesis. A data-driven model is assembled, but there may be unresolved relationships. The machine asks the human user for help.
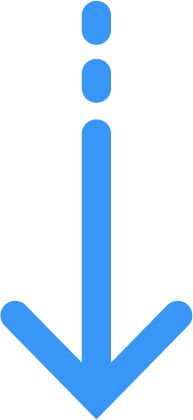
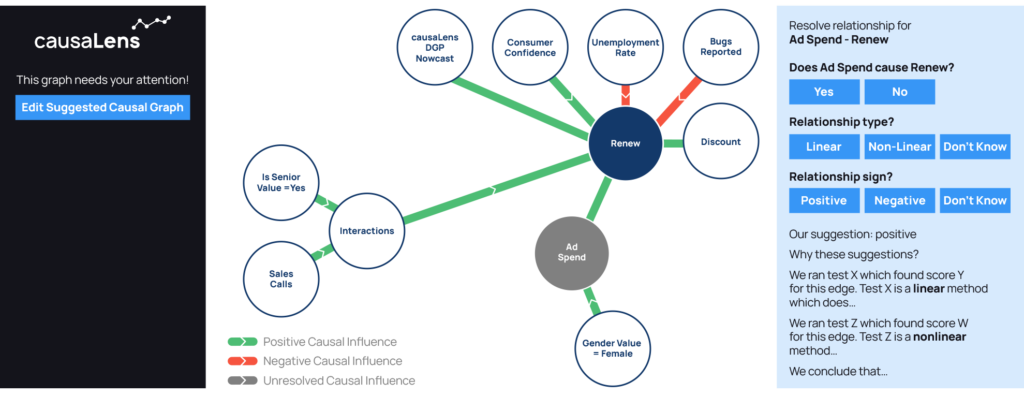
Back to the human user who can accept/decline suggestions and further refine the model by adding information about the nature of the relationships (for instance whether the relationship is positive/negative and linear/non-linear).

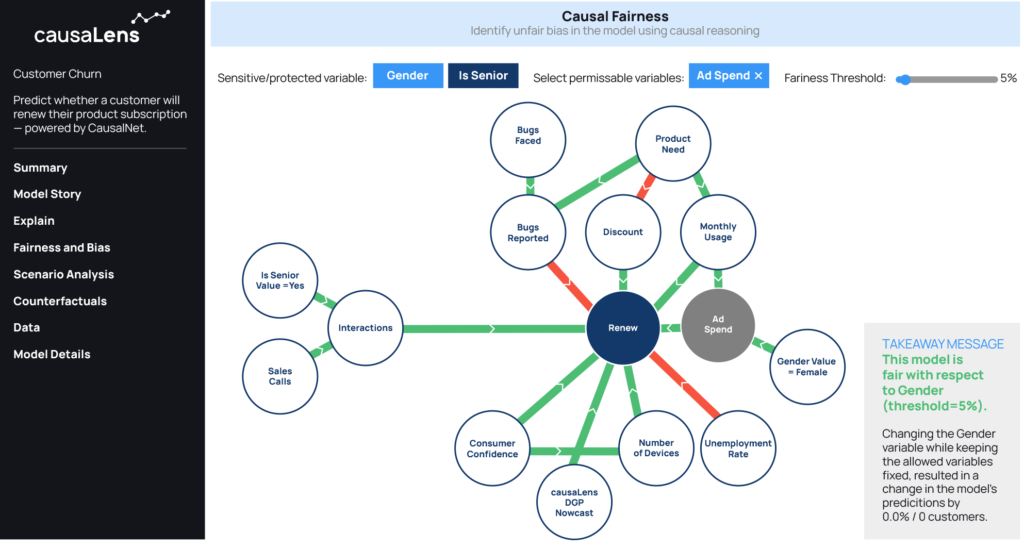
Business users can also check that sensitive information is not inappropriately influencing decisions, by running counterfactual fairness tests. Causal AI will suggest appropriate fairness criteria based on its understanding of the business context.
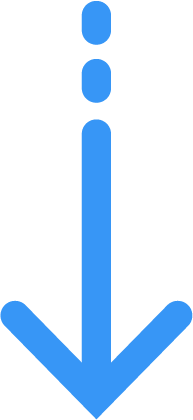
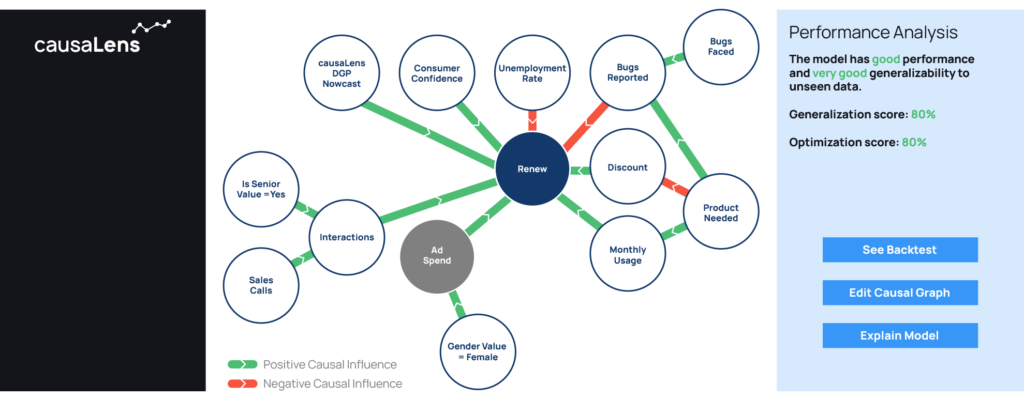
The result is a model that is both data-driven and closely aligned with the business users’ expertise, common sense, and constraints. Key decision-makers who are ultimately responsible for applying the model trust it and so are comfortable signing off on it.
Watch our webinar on human-guided causal discovery to learn more about how this works in practice.
What’s the impact of using a human-guided approach
to Causal AI model-building? We estimate an increase in development speed of 10X and a comparable acceleration of model risk governance reviews.
Key 2. Design AI systems that go beyond predictions to offer prescriptions
Causal AI directly enhances decision-making. It is equipped with humanlike reasoning capabilities that go far beyond making narrow predictions.
Causal AI can run virtual A/B tests, enabling users to run high volumes of experiments without any costs or delays. Causal AI models generate recommendations and interventions that can be scrutinized by business users or directly pushed to software systems to execute. Business stakeholders can explore data-driven scenario analyses and explore what-if questions, to support ideation and hypothesis-testing.
Let’s return to our customer retention example to illustrate.
Conventional AI treats this as a predictive problem— which customers are likely to churn, and which are likely to renew? The problem with this approach is that the likely churners may be totally unpersuadable! You could spend your entire marketing budget trying to persuade them, and it could have zero impact! Indeed, Harvard Business School research indicates that typically only 50% of the most likely churners are persuadable.
We advocate treating churn prevention not as a predictive problem but as an exercise in influencing— which customers are most persuadable, and what’s the best way to persuade them?
Causal AI can run virtual experiments to pinpoint which customers are most persuadable. Virtual experimentation is combined with various constraints— again supplied by domain experts from the business— such as budgets, strategy, and costs associated with an intervention, to generate personalized or segment-level recommendations. Scenario analysis enables marketers to explore how different marketing campaigns and strategies will influence retention rates, and high-level KPIs like revenue uplift or active usage.
The result is that sales and marketing teams can focus their efforts on the highest-value targets with the most effective outreach.
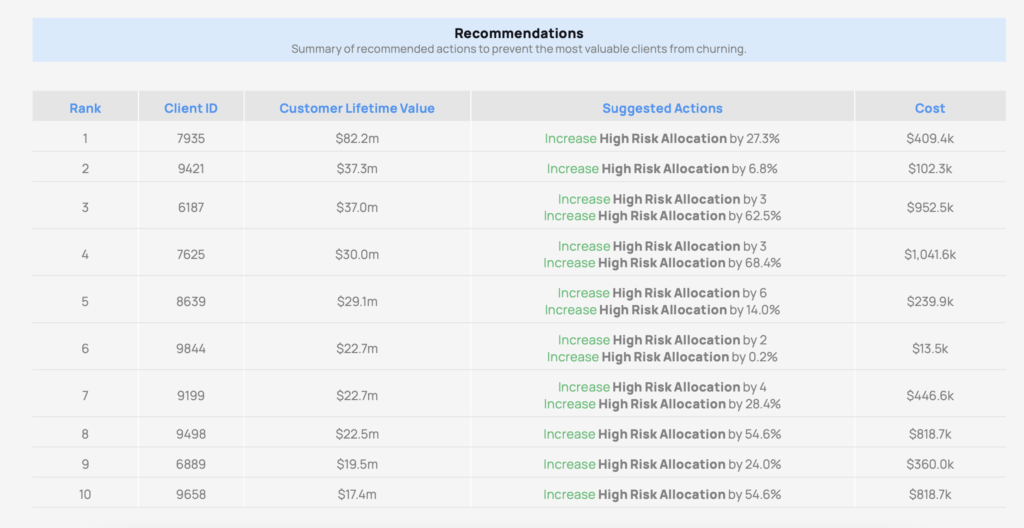
causaLens’ customer retention decisionApp for wealth management. Causal AI provides recommended interventions for each customer, sorted by order of urgency and factoring in lifetime value, and the cost of the recommended action.