Customer Case Study: Reduction in Downtime
Oil Services Company sees $30mn in annual value through reduced downtime
Leading Oil Services Company
Oilfield Services
Digital twin of drilling systems
Downtime drop yields $30mn annual value
The Challenge
Mid-run failures in drilling equipment were notably impacting the bottom line for our customer, one of the world’s largest oilfield service companies. These failures resulted in downtime, significantly increasing the cost of completing jobs for customers and impacting both customer satisfaction and the company’s reputation.
Increasing the rate of penetration can increase the number of mid-run breakages, which are incredibly expensive and very time-consuming to fix. The customer came to causaLens with this problem and wanted to develop a digital twin of drilling runs to understand the trade-off between the rate of penetration and the likelihood of a fault, given certain run parameters.
The customer had previously used traditional ML methods to predict the likelihood of failures but could not explain why a breakage was expected and, more importantly, actions that could be taken to prevent future failures.
-
1Inefficient Planning Leading to Increased Breakages
Maximizing drilling rates is a top priority, but an increased rate of penetration = increased mid-run breakages
-
2No Expert Knowledge in Traditional ML Models
Expertise of oilfield operators can’t be used in other AI approaches
-
3Correlation-Based ML Falls Short
Traditional ML methods only predict breakages but fail to explain why they occurred and actionable steps for prevention
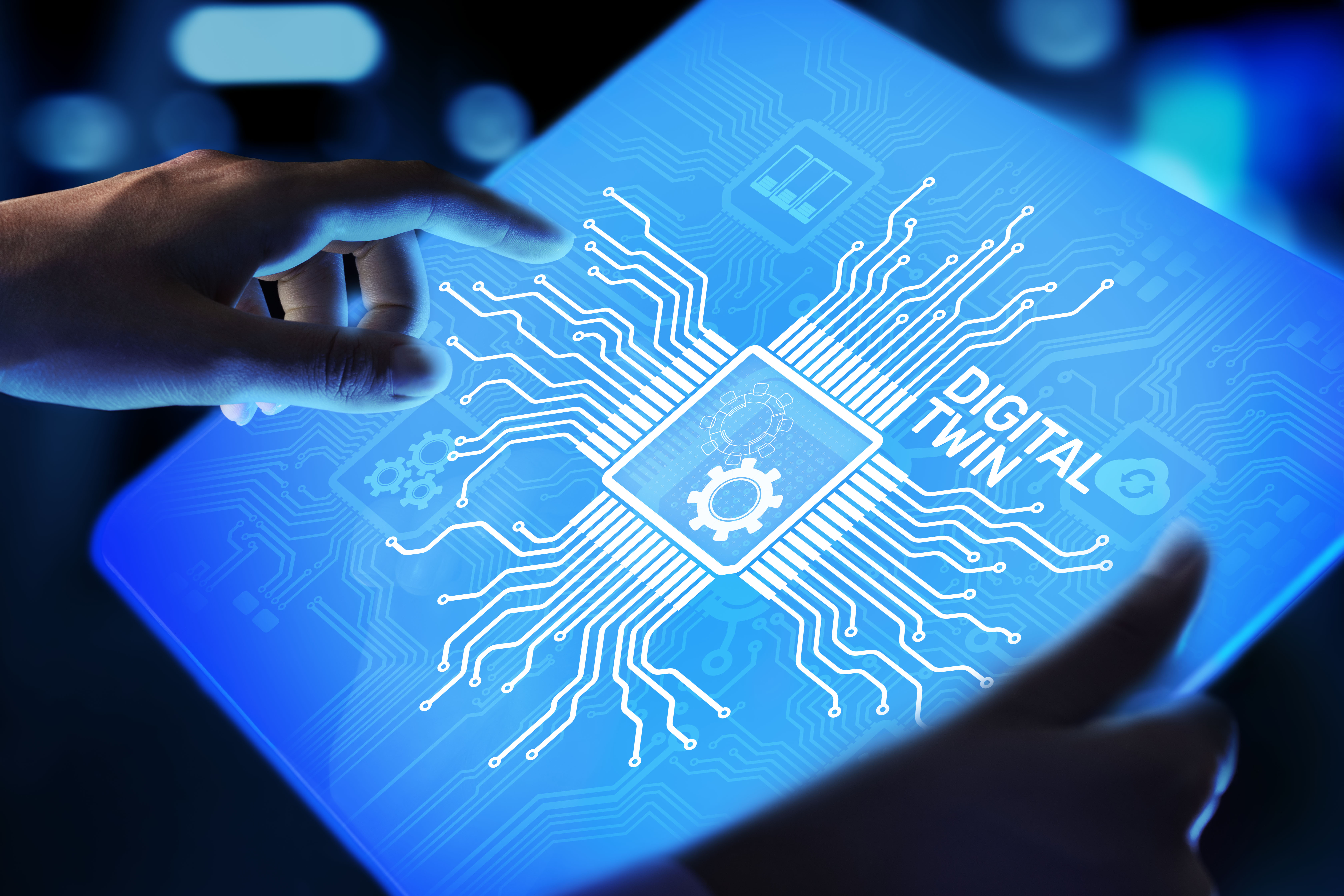
The solution
The customer was familiar with using causality for other problems, but they typically took a manual, hand-drawn approach to create causal graphs and models. They realized the lack of scalability with this approach and that they were missing key insights that lived within the data.
- causaLens offered an automated, scalable end-to-end causal AI approach that used a combination of domain-expertise and data-driven causal discovery to accurately represent each run digitally.
- The solution allowed the customer to ask key ‘what-if’ questions for all of the drilling runs to understand actions that could be taken to optimize each run.
Results and Benefits
The causaLens solution allows the customer to access each drilling run through a custom interactive application that we call a decisionApp. The app enables the customer to ask ‘what-if’ questions and see how changes to run parameters would affect the likelihood of equipment breakages and the rate of penetration.
This capability allows customers to evaluate different scenarios and plan their drilling runs in a way that minimizes the risk of breakage and optimizes the completion time.
The solution plays an important role in increasing efficiency and improving profitability with a more stable drilling process.
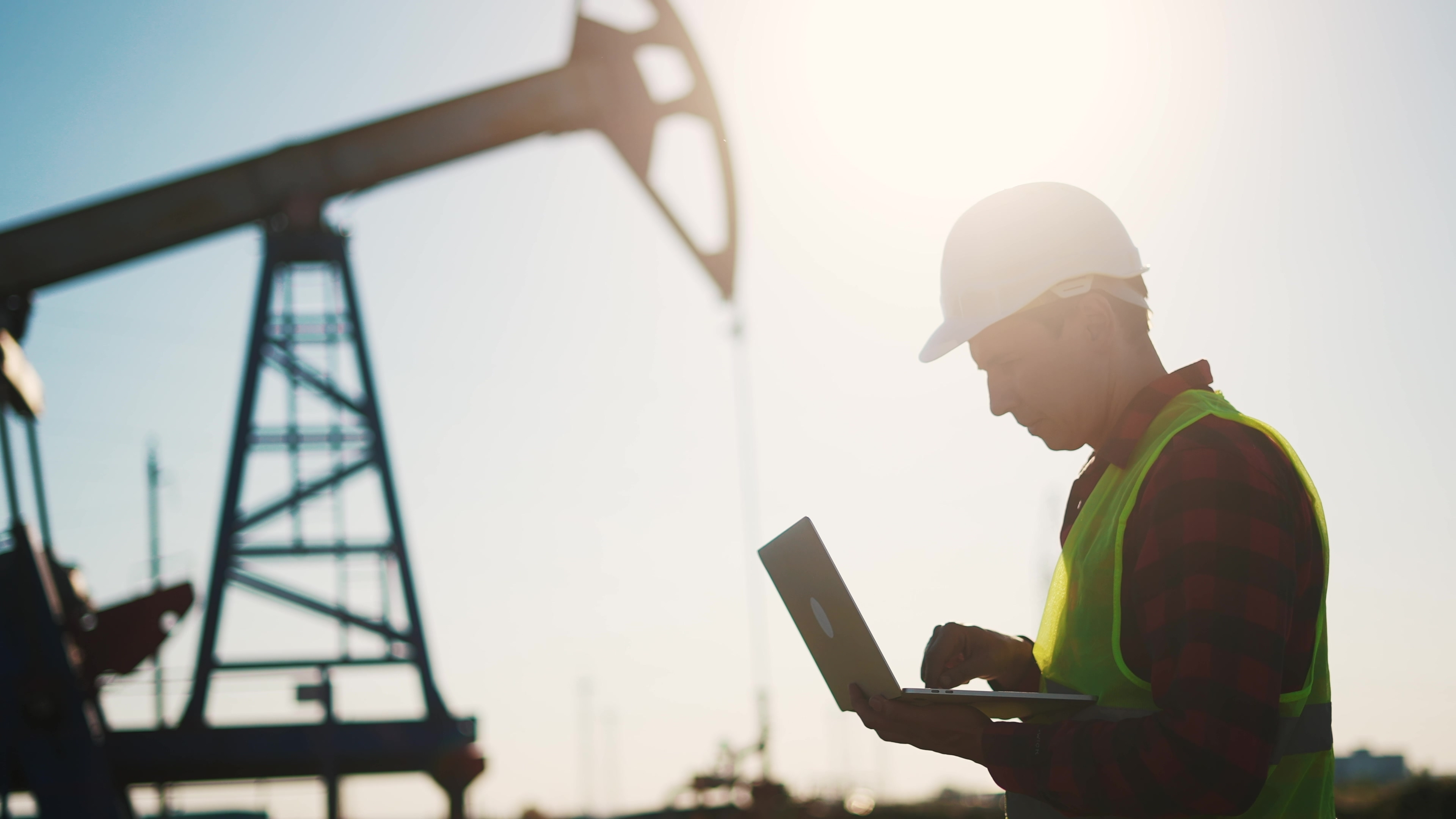