Customer Case Study: Manufacturing Downtime Reduction
$10bn Metals Enterprise sees an expected return of $4M from maximal throughput while reducing downtime
$10bn Metals Enterprise
Metals & Mining
Manufacturing downtime reduction
$4M ROI per year by maximising throughput while reducing downtime
The Challenge
One of the world’s largest mining and metals companies wanted to optimize one of their manufacturing processes by understanding what drives different types of faults and receive recommendations on optimal operating conditions.
A key driver of operational efficiency was the electrical amperage of the processes. Maximizing amperage leads to a maximal throughput but also a higher probability of faults and therefore downtime. The client wanted to understand the right balance in these operating conditions to reduce these faults and maximize throughput.
The customer wanted to better optimize one of their manufacturing processes to ensure maximal throughput while reducing downtime.
However, traditional, correlations-based predictive maintenance techniques have 3 key limitations:

Correlation, not causation
They simply learn past correlations, not cause-effect relationships, making it impossible to trace back the root cause(s) of different failures

Do not capture engineers' rich domain knowledge
Domain experts speak the language of cause-effect & structural relationships between variables, not the language of historical patterns and correlations

Focus on prediction, not next best action
Correlations-based approaches often help with predicting but struggle to offer actionable insights for optimal operating conditions
Solution
Using our platform, decisionOS, the client was able to:
- Merge domain expertise and data-driven causal discovery methods to discover the cause-effect relationships that lead to the machine breaking down or operating less efficiently using our human guided causal discovery framework
- Develop causal predictive models that go beyond past correlations and understand cause and effect relationships in order predict inefficiencies and faults using our structural causal model
- Trace back the root causes of inefficiencies & faults using our root cause analysis engine
- Receive optimal interventions and operational settings that maximize throughput and reduce downtime using decision intelligence engines such as algorithmic recourse
- Empower their engineers to harness all these insights through intuitive decisionApps, deployed in the platform
Results and Benefits
The client was able to trust the insights & decision workflows developed in our platform, deploying them in production. Our Causal AI methodology was key to unlocking the trust from the engineers & executives.
This lead to:
- Robustly predicting and explaining failures in real time empowers engineers to act quickly
- Identifying common root cause paths allows to unearth inefficiencies in the current process and decide where to invest in new tools and machinery
- Expected ROI of $4M per year
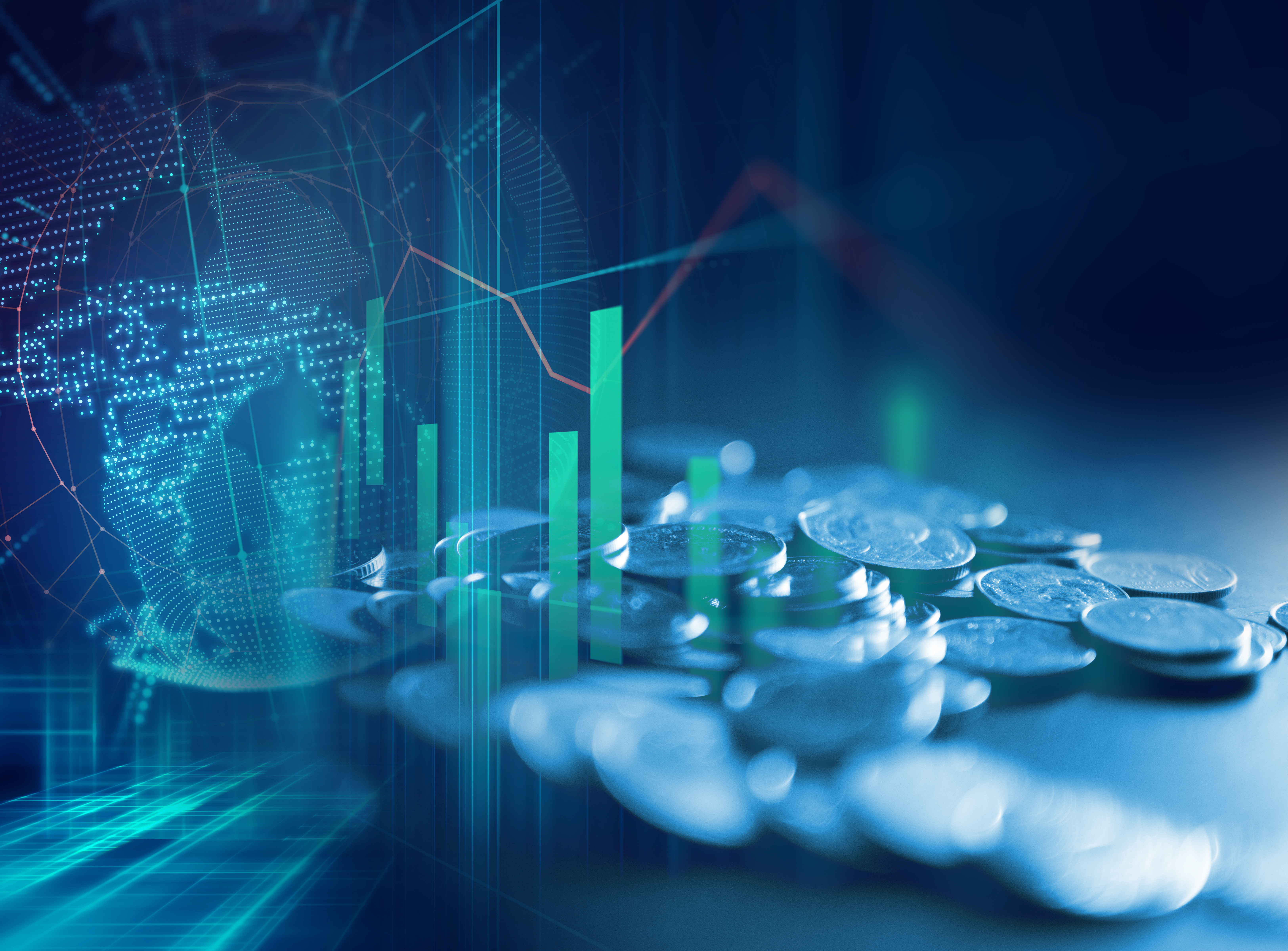