- Blog
Incoming EU regulations on Enterprise AI: why you need to prioritize the adoption of Causal AI
Incoming EU regulations on Enterprise AI: why you need to prioritize the adoption of Causal AI
The EU has just released a new regulatory proposal meant to tackle the usage of ML/AI models within the union. Any company that deploys models that are considered high risk will fall within the scope of the regulations.
One of the requirements is having human oversight. For humans to truly appreciate how AI works, there needs to be a layer of transparency — essentially looking under the hood of algorithms. A lot of existing AL/ML solutions are black-box solutions, which means organizations are unable to connect the dots as to how these machines reach certain decisions. This can lead to disastrous mis-performance and unfair outcomes. The regulation is pushing organizations to become more accountable for the AI machines they are deploying, which is pivotal as AI plays an increasingly significant role in business decision-making. Causal AI could be the key to providing transparent and accountable decision-making AI for businesses.
The significance of the regulation for businesses
The proposals would be a major step-change for the industry. They are putting in place a set framework which businesses need to adhere to when using AI models: organizations will now have to become more critical of AI models to mitigate risks. Pseudo-explainability solutions — like Shapley values — aren’t expected to be accepted by regulators.
Explainability
It’s important to emphasize what we mean by explainability. Currently, there are tools available to attempt to inspect black-box AI systems, but these are ex post pseudo-explainability tools: we can try to inspect the answers that the models are given after they are trained, but without any assurance that they will always give sensible answers to the same question. Next-generation models should be more scientific in their reasoning. They should have an embedded set of core principles and assumptions that are never violated: explainability should be an ex-ante concept.
An AI system is truly explainable if its decisions or predictions can be explained to, and understood by, humans — and the decisions cannot violate its core set of assumptions and principles. One consequence of the lack of ex-ante reasoning is that many black-box AI systems can be victims of adversarial attacks — whereas by exploiting the lack of robustness in the models, bad agents can find simple loopholes to change the system’s behavior.
Another consequence is that they can make biased decisions by accident: using zip code to make credit decisions may seem innocent, but in reality, it will act as a proxy for ethnicity.
Causal AI is truly explainable. Causal diagrams represent relationships between variables in data, in an intuitive and efficient way. This tells us more than Shapley values because we know that these relationships are causal and we can be confident that they will be maintained in production.
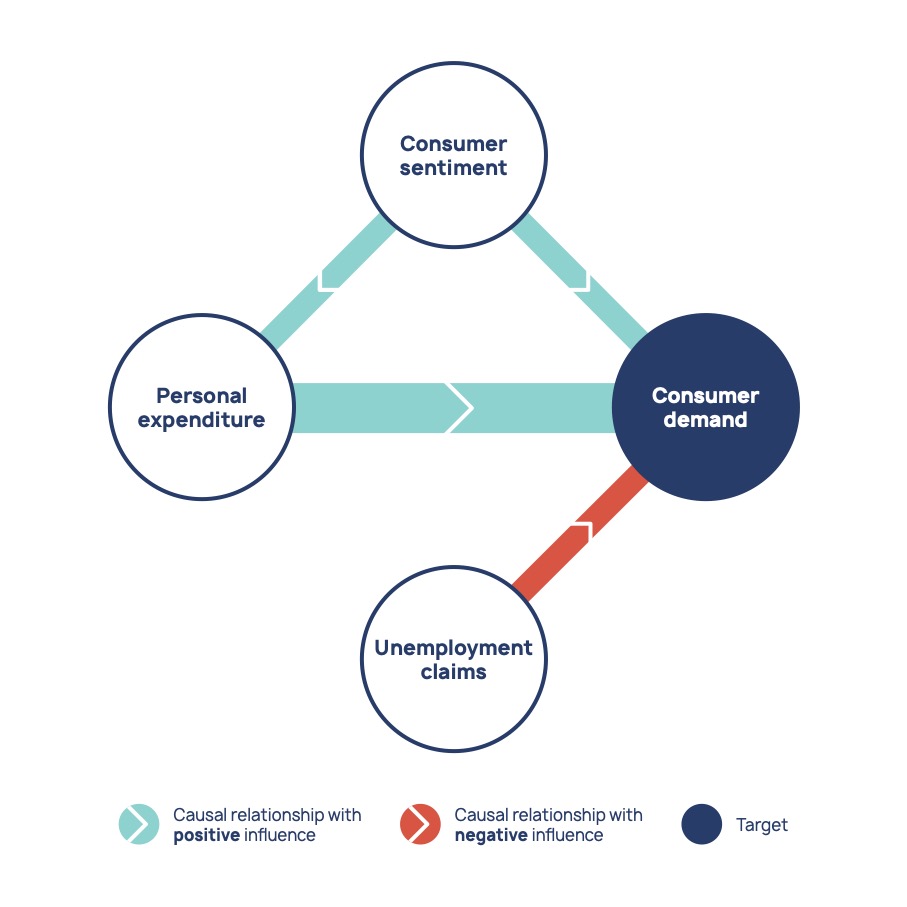
Causality and Causal AI: more trust, less bias
What causes AI systems to become biased? Algorithms are a product of the environment and society in which they’re trained. They learn human biases that are implicit in the data they’re exposed to, and their usage may reinforce existing biases. To avoid bias, machines need to be capable of understanding cause-and-effect relationships that go beyond historical data sets, so organizations can change certain elements of the algorithm to adjust the outcome. Focusing on causes instead of correlations provides a different approach to algorithmic bias that promises to overcome the problems that conventional ML runs into.
Business leaders should call for causality
While the new regulatory framework may seem overbearing at first and can indeed become costly, business leaders also need to recognize that they are directly or indirectly liable for the performance of their AI systems. Thus the main concern here shouldn’t be about compliance, but rather ensuring that their businesses are protected and that they can fully trust their AI systems.
In the current generation of AI systems, explainability, trust and fairness come at the expense of accuracy. More explainable models, such as linear regression, do not have the power of more complicated, inscrutable models, such as deep neural networks or ensembles.
But across organizations, there is a very high premium on AI systems that make accurate decisions: from algorithms that optimize an energy grid, through to an AI model making medical diagnoses. Compromising on model accuracy can have severe commercial and ethical consequences. Business leaders should look out for and leverage next-generation technologies, such as Causal AI, that facilitate explainability without sacrificing accuracy and performance capabilities.
Interviews with the press
causaLens Director of Data Science, Dr. Andre Franca, was interviewed by the press as an expert on the topic. Below are some of Andre’s quotes. Click on the quotes to see the full story, and browse our FAQs if you have any remaining questions.
The Wall Street Journal
BBC News
Fortune