The Real AI Revolution: Machines That Learn Like Scientists
Machines That Learn Like Scientists
Businesses in every sector have great hopes that artificial intelligence (AI) can unlock value. They dream of moving beyond patchworks of business intelligence, currently dependent on clumsy manual-intensive processes, towards AI decision making that is truly transparent, adaptable, automated and data-driven. Instead, businesses have been sold statistical ‘curve-fitting’ platforms under the banner of machine learning (ML), increasingly conflated with AI itself. And they are now finding that the methods these platforms use seriously fail when applied to their highest-value problems. Fitting curves to data, after all, is only reliable for finding patterns in static conditions, that do not reflect the rapidly changing supply and demand dynamics of today’s world. Truly intelligent machines must do better.
ML has wrongly become synonymous with AI. We must shake off this misconception to start the real AI revolution. Data science must forgo its reliance on curve-fitting ML and return to its roots; to put the science back into data science. A growing number of leading scientists — from Turing Award-winning Professors Judea Pearl and Yoshua Bengio, to Professor Bernhard Schölkopf, Director of Germany’s Max Planck Institute for Intelligent Systems — are advocating for the development of a new science of causality, that goes far beyond statistical pattern-matching. causaLens is a major contributor to this new science of causality. And it is our mission to help organizations of all types to benefit from it.
ML predictions: overfitted and undermodelled
Whilst the exponential increase in computational power has propelled the usage of ML techniques, such as deep learning, these techniques have no comprehension of causality. Fundamentally, all of the latest ML methods rely on building models from statistical associations present in historical data, without first understanding if they are meaningful.
For example, consider the infamous observation that the butter production of Bangladesh is correlated to the returns of the US stock market. We should not place any trust in such a surprising correlation without a model that demonstrates why Bangladeshi butter production influences American stocks.
Such a model would be able to address questions like: “would the American stock market crash if Bangladesh ceased producing butter?” By using traditional ML we would simply obtain a nonsensical answer because ML is ‘model blind’. ML methods are completely at the mercy of whatever statistical associations lie within the data sets that they learn from.
Of course, the statistical techniques ML uses are based on rigorous mathematics. However, like all mathematical
techniques, they must be applied under appropriate conditions in order to produce desired results. Building predictive models blindly from statistical associations only makes sense for data generated by systems that are largely static: where the statistical associations between variables remain the same. Unfortunately, in the real business world they are always changing. As a result, as for Bangladeshi butter production and American stock returns, many historical correlations do not represent relationships that are really driving the behaviour of the system. Such unstable correlations simply have little, or no, predictive power. But ML treats all correlations on an equal footing. This leads to ‘overfitting’: models that generate poor predictions.
ML applied to dynamic business systems makes an assumption that just does not hold: that strong historic correlations will always yield good future predictors. There are, of course, many techniques that have been developed to reduce ML overfitting. Nevertheless, whereas these techniques simplify ML models, they still cannot tell true causal drivers from correlations.
ML makes an assumption that does not hold: strong historic correlations will always yield good future predictors
causaLens pioneered automated machine learning (AutoML) for time series with the strongest overfitting protection in 2017. Others soon followed. This marked a step change in predictive capability. AutoML is well-named: the work of human data scientists applying ML was largely automated, saving time and money. But our ambition for AI is greater still. We are determined to address the flawed assumption that correlations are enough to make predictions – an assumption that AutoML currently depends on. AI needs new approaches that do not just identify correlations but interrogate them for predictive power. The curious case of butter production correlated with American stock returns provides a clue as to what such an approach might look like.
Intuitively, we identified a way to challenge this relationship by asking: what if butter production ceased? We can generalize this concept into a wider tool to understand relationships in observational data: the ‘counterfactual’ – in this case, hypothetically stopping butter production. If we could establish that American stocks would remain largely unaffected, we can protect ourselves from making naïve decisions based on this correlation.
Predicting the effect on stock returns if butter production were forcefully ceased – without actually shutting down butter production — is a causal inference problem. Robust causal inference methods simply do not exist in current state-of-the-art ML platforms. We need new tools and principles that enable us to harness powerful ideas from causality, such as counterfactuals.
AI needs to understand causality
By thinking in terms of counterfactuals, machines begin the journey towards building models based on scientific principles rather than simply on statistics. A correlation invites us to pose a scientific hypothesis of a real cause and effect relationship between the variables. If we then have the ability to argue using counterfactuals, we can understand whether these relationships really do have predictive power, as opposed to being spurious -appearing just by chance – or, crucially, whether they are the result of confounders: where two or more variables have an unobserved common cause.
Confounders are ubiquitous but often subtle enough to lead businesses astray. For example, they may both be driven by healthy global export volumes. But using butter production in place of the confounder, global trade, is a profound mistake: another variable, like milk prices, could affect butter production without influencing global trade. As global trade, not butter production, is the real driver, the change in butter production due to milk prices would have no effect on stock returns. Thinking in terms of the counterfactual challenge “what if butter production ceased?” allows us to tackle even this complicated confounder. And by avoiding spurious correlations and confounders, machines keep to the path of rigorous scientific modelling where variables have real causal links between them.
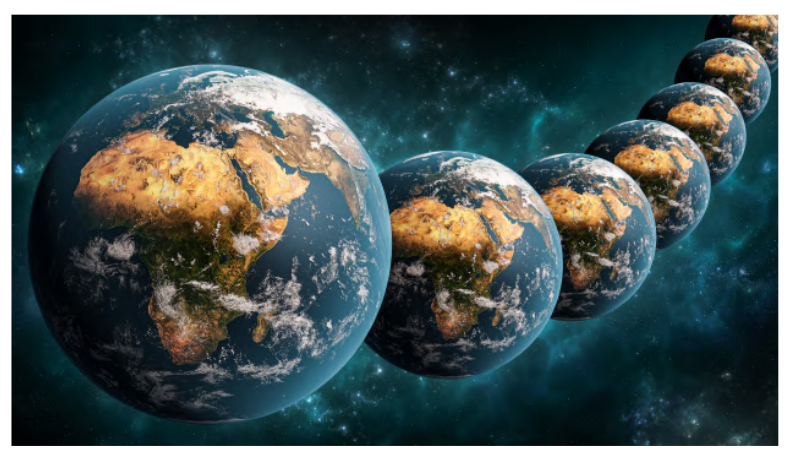
Machines should not just blindly follow statistical patterns but apply the scientific method in how they learn. By understanding causality, they become more than simply machine learners, they become machine scientists. Machine scientists aim to build models based on causal relationships within the system, experimenting with the data to understand the real drivers of behaviour, and discarding spurious and confounded correlations. At the same time, these machines can harness the power of big data and automation to conduct each of these steps much more quickly than humans do. They will unlock a new era of discovery in data science.
Causality is the difference between machine learners and machine scientists
Crucially, AI that understands causal structures can pose, and answer, counterfactual questions about the business systems it is modelling, such as “if we increased prices, what would the impact be on sales?” Incorporating the results of these artificial thought experiments makes the models more robust to a range of possible future scenarios. And with the processing power of large-scale computing, the number of worlds machines could imagine would far exceed human capabilities. As a result, whether due to sudden health crises or climate change, businesses could still trust these models when the world inevitably changes. Such robustness is extremely valuable. Causal models do not just provide protection against the uncertainties of rapidly changing market dynamics but empower companies to seize the inherent opportunities that come with change. ML, for all its achievements, does not have the intelligence to address some of the highest value business problems because it lacks any concept of causality. For AI deserving of the name, we must build Causal AI.